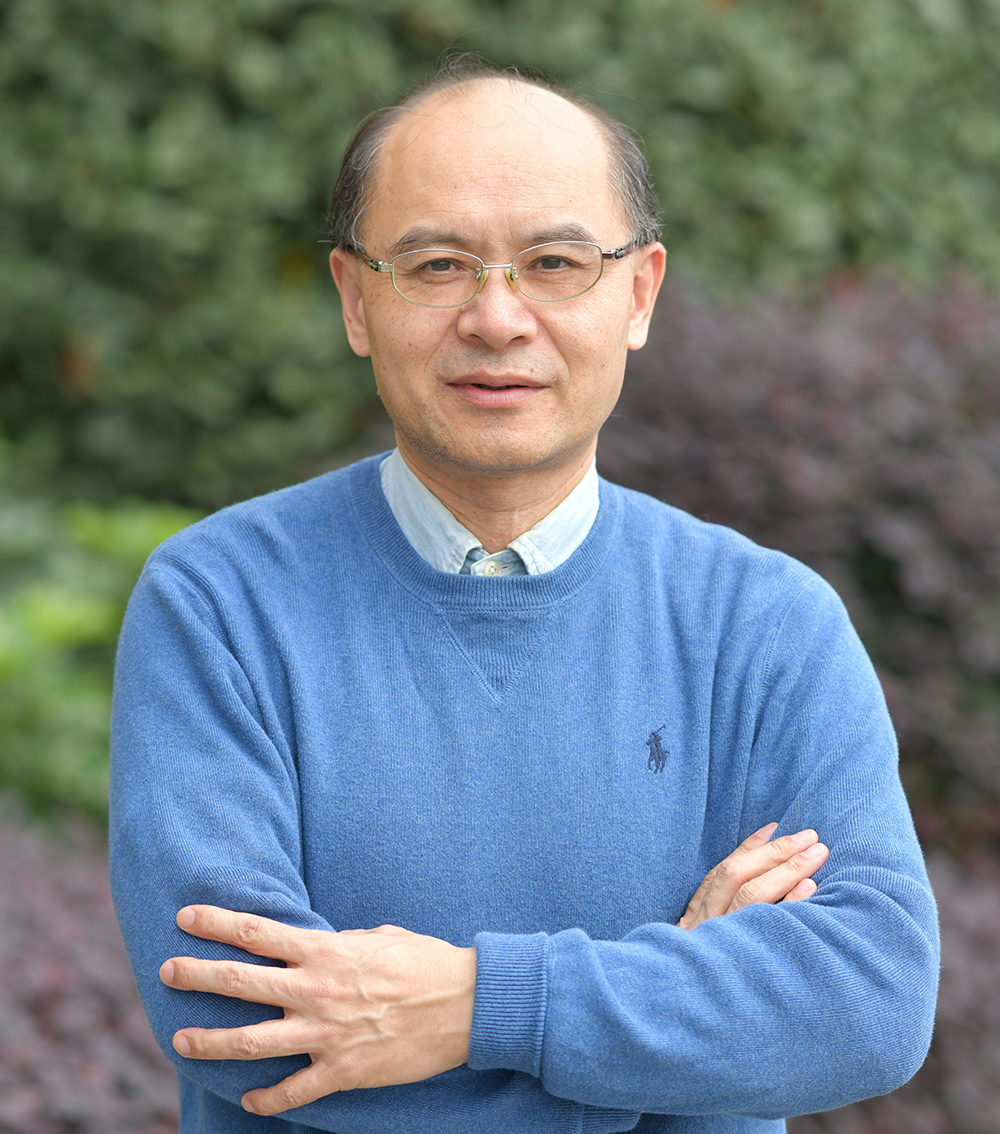
Editor’s Note: With funding from the Shanghai Municipal Science and Technology Commission (Project Number: 22DZ2304300), The Paper and the journal "World Science" have collaborated to report on the award-winning achievements recognized by national and municipal science and technology awards in Shanghai.
This report focuses on the first-prize project of the 2020 Shanghai Natural Science Award, titled "Dynamics-Driven Research on Data Science Theory and Methods." This award was received by the research team led by Researcher Chen Luonan at the Chinese Academy of Sciences' Center for Excellence in Molecular Cell Science.
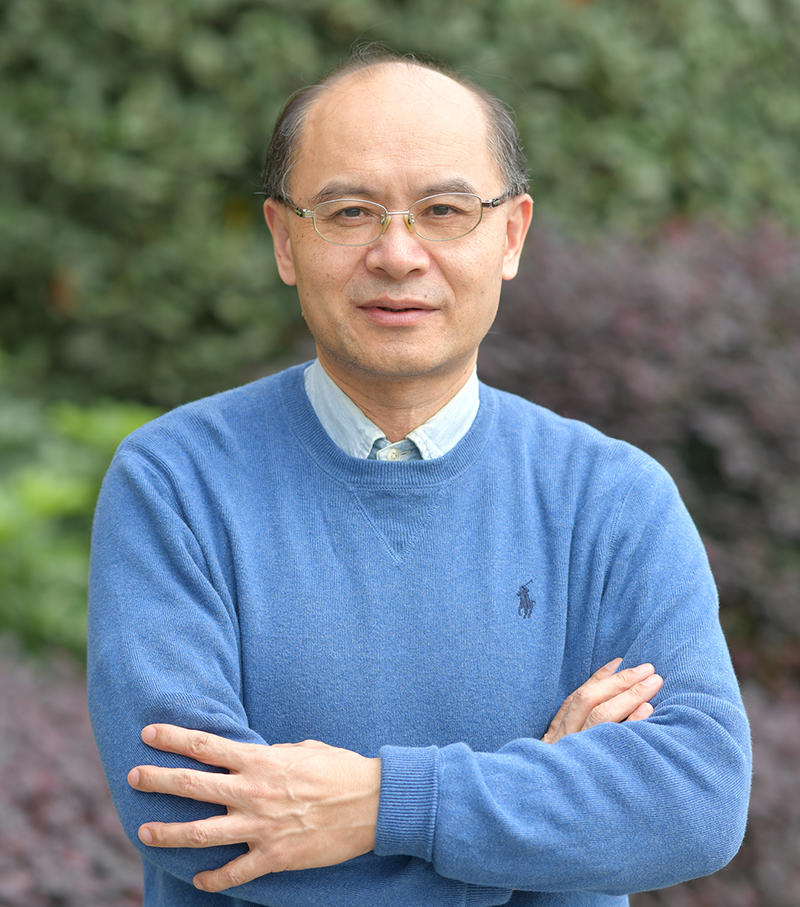
Researcher Chen Luonan from the Center for Excellence in Molecular Cell Science at the Chinese Academy of Sciences.
“You cannot step into the same river twice.” The philosopher’s words dramatically describe a world in constant flux.
How do we describe the state or dynamics of an object?
In 1687, British physicist Isaac Newton outlined three fundamental laws in his work, "Philosophiæ Naturalis Principia Mathematica." Thus, when an object possesses mass, its velocity, acceleration, and the forces acting on it can be clearly defined.
The study of the changes in the motion of objects and their driving forces is known as dynamics. Dynamics models, as a theoretical framework, are used to describe the states and behaviors of objects or particles within a system.
But can we find “dynamical” characteristics or patterns in a data set that lacks mass or within abstract, complex systems?
More importantly, what use would that be?
“We have advanced this field. We have introduced dynamic ideas and methods into data science, which is primarily statistical in nature,” said Chen Luonan, a researcher at the Center for Excellence in Molecular Cell Science at the Chinese Academy of Sciences.
Previously, his research team, which includes Fudan University Professor Lin Wei, South China University of Technology Professor Liu Rui, and Chinese Academy of Sciences Academy of Mathematics and Systems Science Researcher Wang Yong, won the first prize for the “Dynamics-Driven Research on Data Science Theory and Methods” at the 2020 Shanghai Natural Science Awards.
They termed their original research system “Data Science Characterized by Dynamics,” which encompasses aspects such as prediction, early warning, causality, and AI (artificial intelligence), and has been applied in fundamental research across various disciplines, including computational systems biology, critical analysis of biological processes, early warning for tumor cell metastasis, and natural disaster alerts.
The Harvard Business Review once published an article claiming that data scientists are the most "attractive" jobs of the 21st century.
As early as 1974, computer scientist and Turing Award winner Peter Naur proposed that data science combines mathematics and statistics, specialized programming, advanced analytics, artificial intelligence, and machine learning with domain-specific knowledge to extract hidden information from organizational data.
“(However) the current data science primarily relies on static statistical laws and lacks dynamic characterization mechanisms,” Chen Luonan pointed out.
He believes that dynamics can reflect the dynamic mechanisms and causal relationships of systems, which are more fundamental aspects.
This precisely highlights the limitations of contemporary data science in addressing many real-world issues, especially in the era of big data and AI.
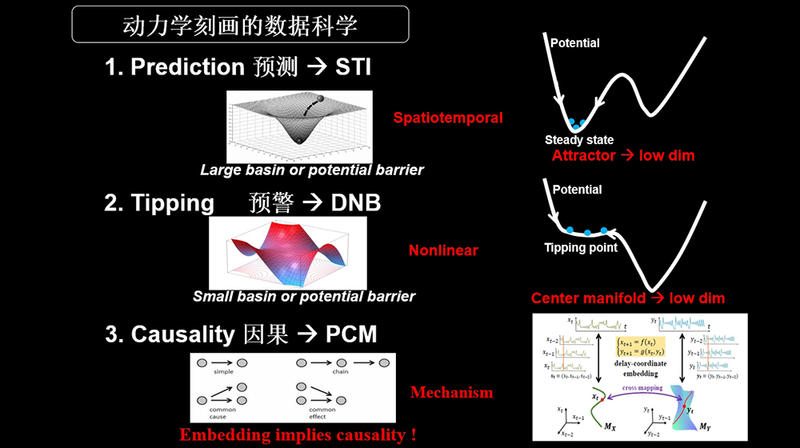
Researcher Chen Luonan from the Center for Excellence in Molecular Cell Science at the Chinese Academy of Sciences has named his team's original research system “Data Science Characterized by Dynamics.”
“Pre-disease” Warning: A “hard brake” before disease worsens
During health check-ups, people often assess their current physical conditions to determine if they are ill.
However, Chen Luonan believes that current check-ups mainly reflect whether individuals are already ill and cannot predict future illness — for example, when someone will become ill, or even, in extreme cases, “how much time is left.”
His team’s series of papers indicate that complex disease processes have a critical state — that is to say, many diseases deteriorate suddenly from an early stage to a disease phase, presenting a “mutation point.”
In their research, patients’ conditions can generally be categorized into three types: “normal state,” “pre-disease state,” and “disease state.” For many chronic diseases, treatment is particularly challenging in the third phase, as it is difficult to return the condition to a relatively normal state, placing it nearly in a non-reversible phase. The pre-disease state represents a critical time point for effective treatment.
“We view complex biological systems as dynamic systems evolving over time, with their critical points corresponding to bifurcation points in mathematical dynamic systems,” said Chen Luonan. However, conventional static comparative studies in medicine often find no significant differences between the pre-disease and normal states. “The static indicators for these two phases typically do not show notable changes, making it difficult to quantify or distinguish the pre-disease state scientifically.”
Theoretically, by constructing mathematical models, one can utilize bifurcation theory to reflect the dynamic critical processes of such complex systems. “But most real-world complex systems do not have precise mathematical models,” he explained. “Without models, we innovate by identifying critical points solely through observational data.”
Using high-throughput technology, Chen Luonan's research team applied critical slowing-down, critical collective fluctuation, and bifurcation theory to propose a novel model-free method based on networks that can detect early warning signals for complex diseases, even with a small number of samples, by examining their “dynamical” critical characteristics and patterns.
Chen Luonan explained that by observing variables measured through high-throughput techniques, they calculate correlations among different variables. If a particular group of variables suddenly exhibits increased correlations and fluctuations, while correlations with other variables decrease, meeting the criteria of all three conditions simultaneously may indicate the dynamic changes of that group of molecular variables as early warning indicators for the complex system transitioning towards a disease state. This group of molecules is termed the leading module or “Dynamical Network Biomarker” (DNB).
“DNB serves as a state evaluator, indicating how far we are from the critical point,” he stated. If a nearing critical point is detected, timely intervention can help prevent the system from transitioning into a disease state.
Currently, this research method has been successfully applied to the diagnosis of tumor cell metastasis, early warnings for autoimmune disease relapses, diabetes critical detection, and investigation of drug antagonism dynamics, and has also been utilized by researchers both domestically and internationally in risk analysis and critical prediction for ecosystems and financial systems.
The paper authored by Chen Luonan's team titled “Detection of Key Nodes and Key Factors in Complex Biological Processes — Early Prediction of Complex Diseases Based on Dynamical Network Biomarkers” emphasizes that a biological system or a complex disease is often modeled as a nonlinear dynamic system or dynamic network. Thus, the development of complex diseases can be seen as the evolution of this complex dynamic system along the time axis.
“A Leaf Knows Autumn”
Time series is a collection of random variables arranged over certain time intervals. Chen Luonan's team published a paper in 2020 indicating that making accurate predictions based on observational data, particularly Short-Term Time-Series (STT) data, is critical to advancements in fields such as neuroscience, atmospheric science, and engineering. However, existing forecasting methods like statistical regression and machine learning require sufficiently long time series measurements and are unable to predict short-term time series.
The aforementioned paper states that high-dimensional observable data within short-term time series contains rich temporal information, which can be used to present and predict the dynamic changes of complex systems. In other words, it is possible to know “a leaf knows autumn.”
However, due to a limited data volume and lack of statistical patterns, “it is essential to ‘unfold’ the temporal information embedded in high-dimensional data, necessitating new theories and methodologies,” Chen Luonan remarked.
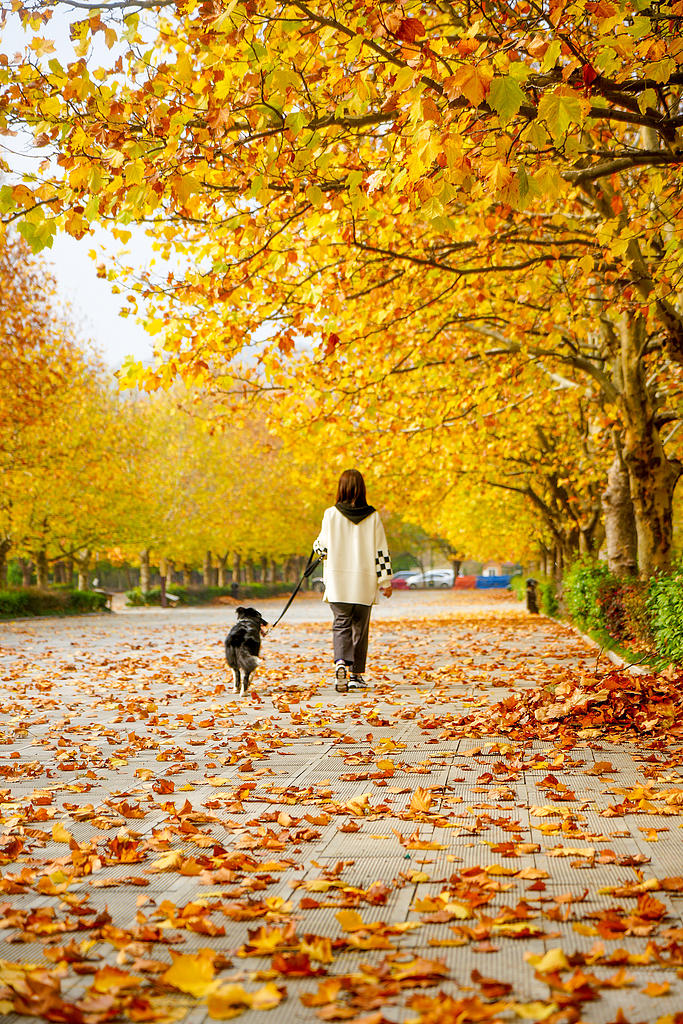
Chen Luonan's team, based on delay embedding theory and generalized embedding theory, established the Spatial-Temporal Information Transformation (STI) equation, which converts the correlation information of high-dimensional variables into the temporal evolution of the target variable. Based on this, methodologies like RED (Randomly Distributed Embedding), ARNN (Auto-Reservoir Neural Network), and ALM (Anticipated Learning Machine) were proposed to carry out multi-step predictions for the target variables, thus rendering more precise short-term forecasts of complex nonlinear dynamic systems.
Unlike traditional statistical machine learning, the STI equation forecasting method originates from nonlinear dynamic system theory, paving a new path for dynamics-based machine learning or deep learning.
Currently, this approach has achieved more accurate short-term forecasts than other methods in predictions involving gene expression datasets, stock data, traffic datasets, and satellite imagery of typhoons.
Chen Luonan indicated that the team is working on enhancing geological disaster warnings by integrating DNB early warning and STI forecasting methods, with plans to extend these applications to even more contexts in the future.
On September 5, 2023, the prestigious academic journal "Proceedings of the National Academy of Sciences" (PNAS) published the latest research on real-time earthquake premonitory warnings, co-authored by Researcher Chen Luonan's team from the Institute of Biochemistry and Cell Biology of the Chinese Academy of Sciences and Liu Rui's team at the School of Mathematics of South China University of Technology. This research achieved 83% true positive and 0.98% false positive rates in its warning accuracy. The detected early warning signal accuracy significantly surpassed that of existing 10 methods, providing an average warning time of 6-7 days in advance. Therefore, this method holds significant practical application and reference value in earthquake disaster monitoring. The results also suggest that strong and weak earthquakes may involve different dynamical factors or mechanisms.
Innovative Research: Pioneering a New Discipline
“The work we are doing now is different from current methodologies,” Chen Luonan asserted. “Our work has developed this field.”
Existing papers on disease early warning mostly utilize case-control studies to observe statistical differences, remaining static in approach. If static comparative studies can evolve into dynamic process-oriented studies, “the information would be more complementary, thus revealing aspects that were previously unnoticed.”
Currently, Chen Luonan's team is also conducting research on prediction and early warnings. He acknowledged that while foundational methods have been introduced, many unresolved challenges remain.
He pointed out that noise interference and the inherent strong randomness of systems pose considerable challenges. Additionally, the proposed prediction and warning methods have limited applicability in real-world scenarios, and achieving greater generalizability remains a pressing concern. “Moreover, accurately identifying DNB from high-dimensional data is also an issue,” Chen Luonan remarked.
He noted, “We have proposed these concepts and methods, and ultimately we need everyone to collaborate to refine them.”
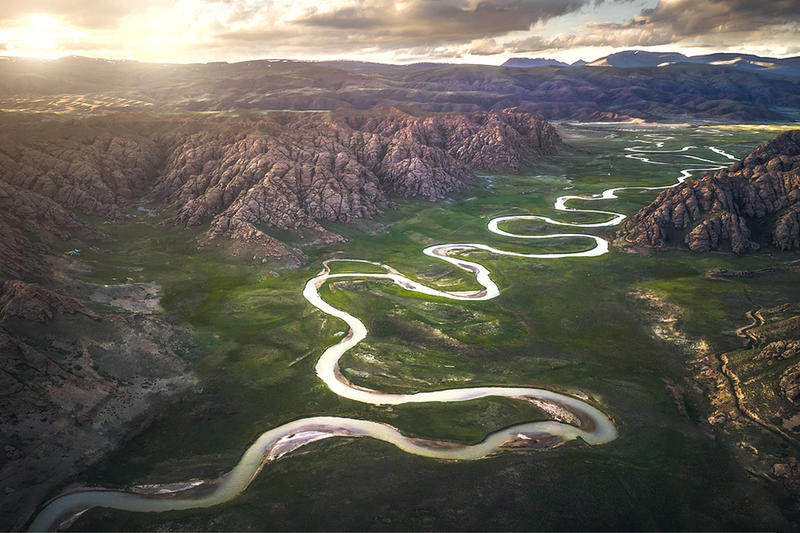
“You cannot step into the same river twice.”
Chen Luonan also revealed that the team has initiated research on "pre-disease" projects and has promoted the establishment of a national science and technology initiative on “pre-disease.”
He explained that the concept of “pre-disease” is a significant principle in traditional Chinese medicine, representing a critical state in the development of diseases, where reasonable interventions during this phase can reverse disease progression. However, the development process of “pre-disease” is notably dynamic and complex, and many aspects of “pre-disease” in traditional Chinese medicine have not been quantified, lacking scientifically meaningful concepts or standards, which severely hinders the objective identification of “pre-disease” and the early diagnosis and treatment of diseases. The research team led by Chen Luonan aims to establish a quantitative representation of “pre-disease,” particularly through the critical theory in the DNB framework, to quantify the pre-disease state, thus enabling a “scientific” understanding of the “pre-disease” concept and providing theoretical grounding and quantitative methods for early warnings and early interventions.
Chen Luonan emphasized the need to “scientize” the pre-disease concept. By “scientizing,” he means converting it to an international standard. This also facilitates the modernization and internationalization of traditional Chinese medicine.
Furthermore, his research team has made significant breakthroughs in developing novel algorithms for identifying causal networks and efficient training tools for impulse neural networks. “Our discipline is highly interdisciplinary, and we welcome talents from various fields to join in its development,” Chen Luonan said.