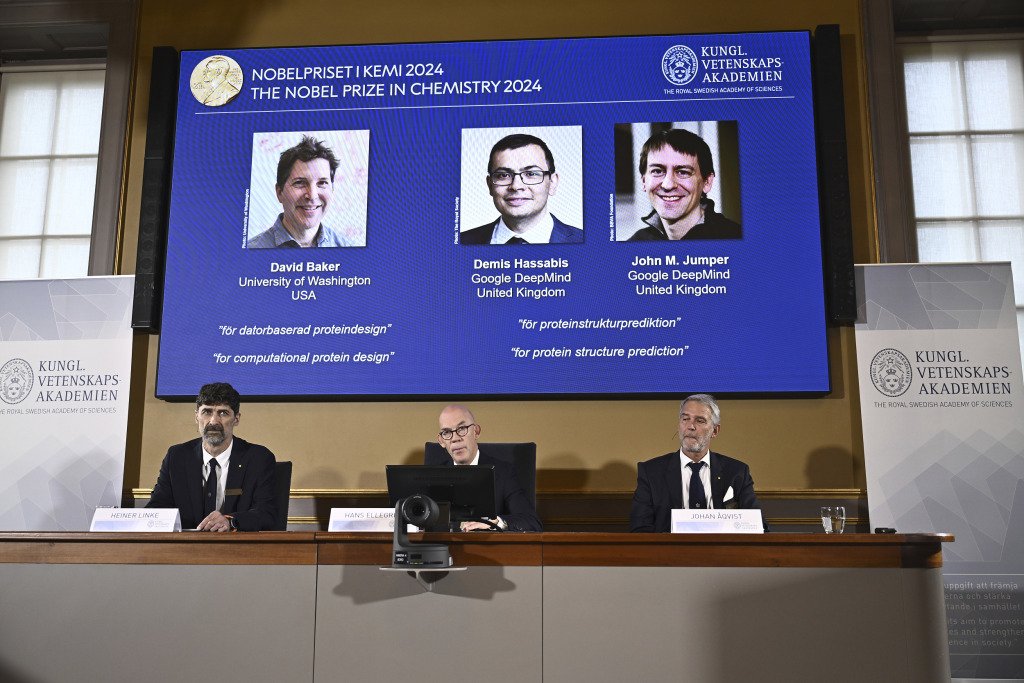
“I've been working on this for my entire career, but I know my peers share the same sentiment — in our lifetime, the 'protein folding' problem is unlikely to be solved, especially the issue of protein structure prediction. Then AlphaFold came along!” On October 9, Professor Ma Jianpeng, a PhD advisor and internationally renowned computational biologist at Fudan University, told The Paper Technology.
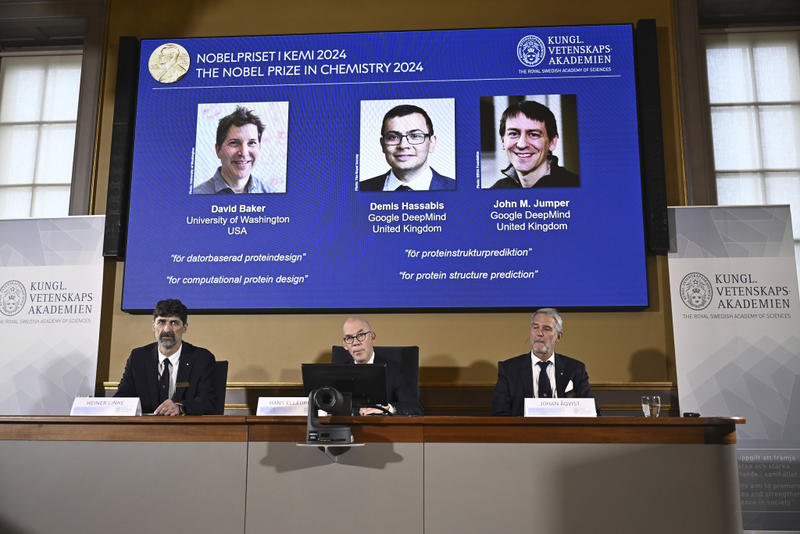
On October 9, 2024, local time in Stockholm, Sweden, members of the Nobel Chemistry Committee, including John Aquist, permanent secretary Hans Ellegren, and chairman Heiner Linke, presented this year's Nobel Prize in Chemistry to David Baker, Demis Hassabis, and John Jumper at the Royal Swedish Academy of Sciences. Visual China photo.
On October 9, local time, the Royal Swedish Academy of Sciences announced the 2024 Nobel Prize in Chemistry, awarding half to David Baker from the University of Washington for his contributions to computational protein design. The other half was jointly awarded to Demis Hassabis and John Jumper from Google's DeepMind in London for their contributions to protein structure prediction.
This is the second Nobel Prize for AI scientists following the awarding of two AI pioneers with the 2024 Nobel Prize in Physics on October 8.
In 2021, Ma Jianpeng's team at Fudan University published the OPUS-Rota4 algorithm, a side-chain prediction algorithm based on backbone structures, which significantly improved the accuracy of protein side-chain structure tests, addressing a weakness of Google's AlphaFold.
It has been reported that this prediction algorithm has "now evolved to OPUS-Rota6, which surpasses AlphaFold by 2/3 in accuracy."
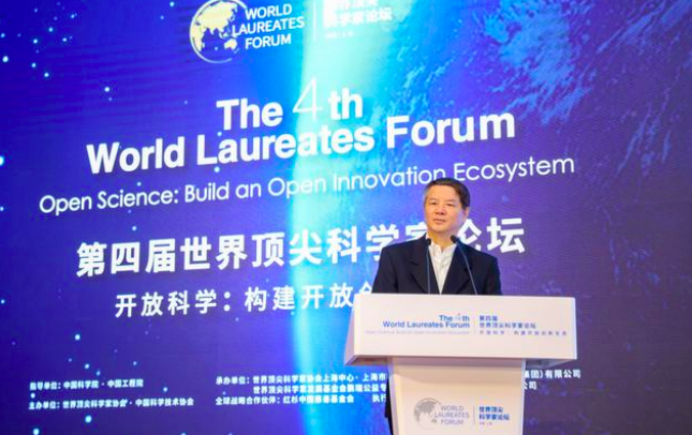
Professor Ma Jianpeng, Dean of the Institute of Multiscale Complex Systems at Fudan University.
Regarding the 2024 Nobel Prize in Chemistry, Ma Jianpeng pointed out that while some may question the awarding of a Nobel Prize to scientists in the AI field, this actually breaks down into two issues: first, should the protein structure prediction be awarded a Nobel Prize; and second, does the contribution of AI in this regard merit a Nobel Prize?
“This is why they received the award. The problem hasn’t been completely solved, but significant progress has been made that exceeds our expectations. It is now usable,” said Ma Jianpeng.
He believes that just as Fudan University announced the launch of at least 100 AI-related courses, AI cannot be overlooked. “You don't necessarily need to be able to write algorithms, but at the very least, you should know how to use it.” He suggests, “Start with the young ones, ensure they understand AI.”
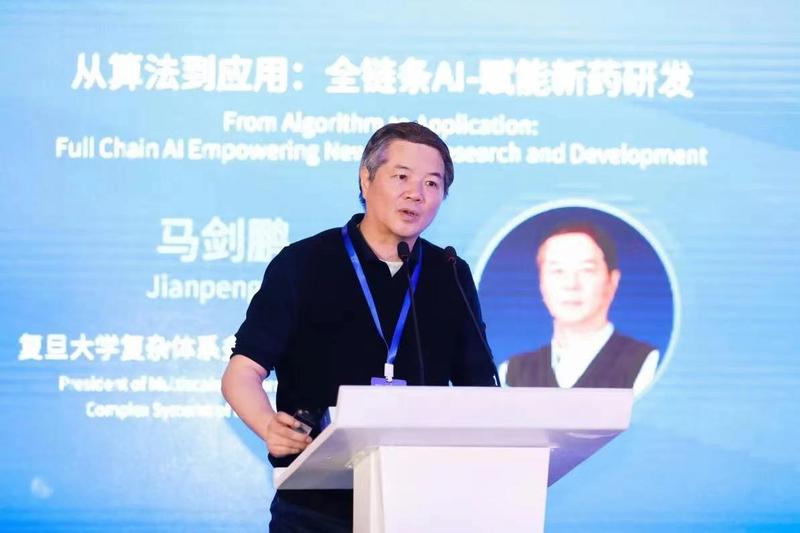
Professor Ma Jianpeng, Dean of the Institute of Multiscale Complex Systems at Fudan University.
He also emphasized, why has Google DeepMind achieved breakthroughs like AlphaFold? This question is of great practical significance for China.
“The Crown Jewel”: An Old and Extremely Difficult Scientific Problem
Why are proteins important?
“Inside every cell in your body, billions of tiny machines—proteins—are working hard.”
Some even say that almost all characteristics of life are related to proteins.
Indeed, proteins are the foundation of every biological process in every organism; they are the cornerstone of life. Without proteins, life simply cannot exist. Their complex and diverse structures correspond to various amazing functions that contribute to the richness of life. The mysteries of life are embedded within their structures.
“When I teach my students, the first thing I explain is why protein structure prediction is so difficult and complex?” Ma Jianpeng said.
Amino acids are linked together to form peptides, and long-chain-like peptides fold into stable three-dimensional structures to become functional proteins. The problem of predicting the corresponding protein's final “folded structure” based on an amino acid sequence is what protein structure prediction entails. This problem is regarded as the “crown jewel” of modern molecular biology.
Ma Jianpeng remarked, “This isn’t a new issue; it’s an extremely old problem, yet it remains very challenging.”
He gave an example: a protein consisting of 100 amino acids is very small. However, if each amino acid has only two states—folded and unfolded (when, in fact, it has infinite states)—then this protein would have 2 to the power of 100 states.
“This number is so astronomical that if we were to exhaustively search for one correct answer using any human computer, it would take longer than the age of the universe. Yet proteins can fold in an instant,” Ma Jianpeng explained.
Scientists determine protein structures using experimental techniques such as X-ray crystallography or cryo-electron microscopy, but these methods are time-consuming and labor-intensive.
Researchers John Kendrew and Max Perutz from Cambridge University made groundbreaking discoveries in the late 1990s, successfully demonstrating the first three-dimensional model of a protein using a method called X-ray crystallography. In recognition of this discovery, they were awarded the Nobel Prize in Chemistry in 1962.
“In 2020, AlphaFold tackled one of the biggest scientific challenges of the past 50 years,” DeepMind’s website states, “achieving a fundamental breakthrough in protein structure prediction.”
To date, AlphaFold has predicted the structures of over 200 million proteins—virtually every protein known to the scientific community—and has helped scientists understand how life molecules interact.
The AlphaFold software has released three major versions. In December 2018, a team using AlphaFold 1 ranked first in the overall standings of the 13th Critical Assessment of Techniques for Protein Structure Prediction (CASP13). In November 2020, a team using AlphaFold 2 again claimed first place in the CASP14 competition.
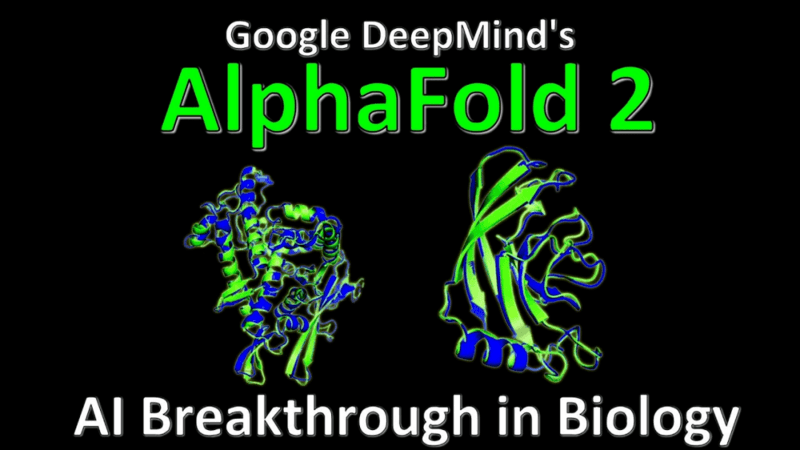
On July 15, 2021, a research paper on AlphaFold 2 was published online in the international academic journal Nature, titled “Highly accurate protein structure prediction with AlphaFold.” John Jumper and Demis Hassabis are co-corresponding authors.
AlphaFold 3 was released on May 8, 2024. It can predict the structures of complexes formed between proteins and DNA, RNA, various ligands, and ions. A related research paper was published the same day in the journal Nature.
According to DeepMind's website, millions of researchers around the world have used AlphaFold 2 to make discoveries in areas such as malaria vaccine development, cancer treatment, and enzyme design. AlphaFold 3 expands its capabilities beyond proteins into a broader realm of biomolecules. This leap can unlock more transformative science, from developing renewable materials and resilient crops to accelerating drug design and genomic research.
Ma Jianpeng said, “In terms of purely modeling protein structures or drug design in the pharmaceutical industry, the accuracy of AlphaFold is far from ideal. Still, it is incomparably better than previous tools!”
Structure prediction is a technique; design is an art
Ma Jianpeng explained that the problem of protein structure prediction actually involves two specific issues—the process of protein folding and the prediction of the final structure. “One issue is how proteins fold. It is essentially about the entire folding process of those 100 amino acids that are linked together. Between the starting point and the endpoint, how does it unfold? That question remains unsolved. However, from a biologist’s perspective, they can bypass the first question; I don’t care how it folds, just give me a protein sequence, and as long as you can tell me the final protein structure, that’s all that matters. The pathway is largely irrelevant,” he noted.
Compared to predicting structures, Ma Jianpeng stated that designing a new protein is more challenging. The former is about solving a problem by predicting the structure of an existing protein, while the latter involves creating a structure that never existed before. “Thus, I always say that folding is a technique, while design is an art.”
One of the 2024 Nobel Prize in Chemistry laureates, David Baker, obtained his PhD in biochemistry from the University of California, Berkeley, where he studied under Randy Schekman, and conducted postdoctoral research in biophysics with David Agard at the University of California, San Francisco. He is now a professor of biochemistry at the University of Washington and the director of the Institute for Protein Design at the University of Washington School of Medicine. Baker's lab develops protein design software and uses it to create molecules to address challenges in medicine, technology, and sustainability. One of his recent endeavors is developing powerful machine learning methods for generating functional proteins.
Baker also holds joint professorships in genomics, bioengineering, chemical engineering, computer science, and physics at the University of Washington. He has published over 600 research papers, co-founded 21 companies, and holds more than 100 patents.
Ma Jianpeng introduced that Baker was among the first in protein structure prediction; long before AlphaFold emerged, he was often a champion at the CASP competitions. His prediction accuracy reached over forty percent. “Baker’s outstanding strength lies in the fact that he not only knows computation and prediction but is also skilled in experimentation and design. He has a strong experimental background, and his team is a very typical blend of ‘wet and dry’ work, which leads to their success.”
In the late 1990s, David Baker began developing computational software called Rosetta to predict protein structures. His research team outlined a protein with a new structure and then let Rosetta compute which amino acid sequence could yield the desired protein. It turned out that Rosetta could indeed construct proteins, as evidenced by the protein Top7 they developed, which closely matched the structure they designed.
Clearly, this software allows people to design proteins as desired for use in drugs, vaccines, nanomaterials, and microscopic sensors.
AI is Everywhere: We Can't Avoid It, So Start Young, Don't Be Left Behind
“I have a viewpoint: I believe that AlphaFold's success may have a more significant impact on AI and computer science than on protein structure prediction itself,” said Ma Jianpeng.
This viewpoint stems from his long-term observation: in 1997, the computer Deep Blue defeated the chess world champion Garry Kasparov. At that time, people thought the world was going to collapse and that computers would disrupt everything, yet nothing happened. People believed that chess was a small enough game to be beaten, but Go could never be defeated by a computer. Then in March 2016, AlphaGo beat Go champion Lee Sedol with a score of 4 to 1, leading to similar concerns about an impending crisis. However, others dismissed it as merely a game. It wasn’t until DeepMind relentlessly developed AlphaFold that the true implications were realized.
“Looking at the field of computer science and AI, if they can solve such a challenging protein structure prediction problem, how significant are things like facial recognition and self-driving? It has truly become ‘AI Everywhere.’ Although AlphaFold isn’t perfect, it is practically usable; it can accelerate scientific research.” Ma Jianpeng stated that the success of AlphaFold has given rise to the term “AI for science,” utilizing AI as a tool to assist scientific inquiry.
At a press conference announcing Fudan University’s 2024 enrollment and training policy, it was declared that starting in the fall semester of 2024, Fudan University would launch at least 100 AI-related courses for the 2024-2025 academic year. “We must start young; you can no longer be ignorant of AI or be unable to use it. Everyone doesn’t need to work on algorithms daily, but all technologists, even those conducting experiments, should at least know how to apply it,” Ma Jianpeng said. The AI algorithms are indeed powerful, and AlphaFold has real practical value now, in contrast to the earlier theoretical pursuits. The presence of technologies like AlphaFold likely allows experimental scientists, including Yan Ning and Shi Yigong, to analyze protein structures more rapidly, though it doesn’t eliminate the need for experiments. “It still cannot replace experiments. As of today, the ‘gold standard’ relies on experiments. If one day the predictive technology becomes accurate enough that the computed structure is definitely correct, that would change the world again.”
Why did Google DeepMind achieve a breakthrough like AlphaFold? Is it purely due to computational power?
Ma Jianpeng asserted, “This question is incredibly meaningful for our country, especially now.”
He indicated that while computational power is important, the key lies in the algorithms.