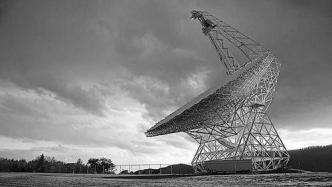
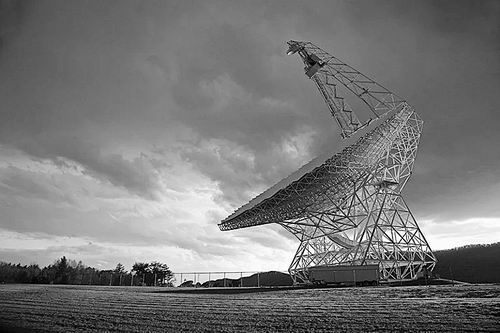
The Green Bank Radio Telescope is one of several telescopes looking for alien civilizations. Credit: Jim West/Alamy
From the hills of West Virginia, USA, to the plains of rural Australia, some of the world's largest telescopes are listening for signals from distant alien civilizations. The Search for Extraterrestrial Intelligence (SETI) is a program to search for man-made electromagnetic radiation.
A study published Jan. 30 in Nature Astronomy describes how machine learning -- a subset of artificial intelligence (AI) -- can be used to help astronomers quickly sift through the vast amounts of data such searches generate. "Machine learning technology has brought SETI research into a new era." Franck Marchis, a planetary astronomer at the SETI Institute in the United States, said.
Big data is a relatively new problem for SETI. For decades, development in this field has been limited by the absence of hardly any accumulated data. Astronomer Frank Drake pioneered SETI in 1960, but subsequent SETI searches have mostly been limited to a small number of stars.
In 2015, Russian billionaire Yuri Milner funded the largest SETI project in history, Breakthrough Listening, in Berkeley, California. The project aims to search for signs of intelligent life on a million stars. Using telescopes in West Virginia, Australia, and South Africa, the researchers looked for radio waves from stars that varied in frequency steadily.
The trouble is, those searches generate reams of data, including false positives from interference from cellphones, GPS and other aspects of modern life.
"The biggest challenge for us when looking for SETI signals is not getting data, but distinguishing signals from humans or Earth technology from signals found elsewhere in the galaxy," said astronomer Sofia Sheikh of the SETI Institute.
Manually reviewing millions of observational data results is impractical. One common approach is to use algorithms to lock in on signals that match what astronomers think are likely alien signals. But these methods can miss potential signals that are slightly different than what astronomers expected.
Machine learning algorithms, trained on vast amounts of data, can learn to recognize the signatures of Earth's disturbances and are therefore very good at filtering out noise. Machine learning is also good at picking up extraterrestrial signals that don't fall into traditional categories, and which might have been missed, says lead author Peter Ma, a mathematician and physicist at the University of Toronto in Canada.
Ma and colleagues sifted through observations of 820 stars through the Breakthrough Listen project, observed with the Green Bank radio telescope in West Virginia. They built machine-learning software to analyze the data, where most of the nearly 3 million signals of interest collected were discarded because they were interference signals from Earth.
Afterwards, Ma manually reviewed about 20,000 signals and narrowed them down to 8 interesting candidates. In the end, however, nothing was found - on the team's second listen, all eight signals disappeared. Still, the method they established can be applied to other data, such as the large collection of observations from the MeerKAT telescope in South Africa; and also to archived SETI data to look for signals that might have been overlooked before.
Machine learning is also at the heart of another SETI initiative launching this month, and AI could also help at other stages of the SETI process. For now, SETI will likely continue to organize the data using a mix of classical and machine learning.
(Original title "Will AI be the first to discover alien civilization? Machine learning algorithms help identify signs of extraterrestrial life")
Related paper information:
https://doi.org/10.1038/s41550-022-01872-z