· Postoperative testing? Needle biopsy? Before surgery, benign and malignant thyroid nodules are difficult to distinguish. The team of Guo Tiannan, a professor at West Lake University, combined high-throughput proteomics with Al technology, and the diagnostic accuracy was increased to more than 85%.
On September 6, researcher Guo Tiannan's team, Professor Li Ziqing's team and clinical collaborators published the paper "Artificial intelligence defines protein-based classification of thyroid nodules" in the academic journal "Cell Discovery", using artificial intelligence Combined with protein expression detection, the benign and malignant thyroid can be accurately diagnosed, thereby helping doctors determine whether the nodule needs to be removed.
In recent years, the prevalence of thyroid nodules has continued to increase, and the prevalence of thyroid nodules in the general population is as high as 50%, that is, 1 in 2 adults may have thyroid nodules.
Data show that only about 10% of them are malignant. That said, most patients with benign thyroid nodules could have chosen conservative treatment rather than excision.
In clinical evaluation, fine needle aspiration biopsy is usually used to obtain tissue samples of nodules for cytopathological diagnosis. Even so, as many as one-third of thyroid nodules cannot be accurately classified.
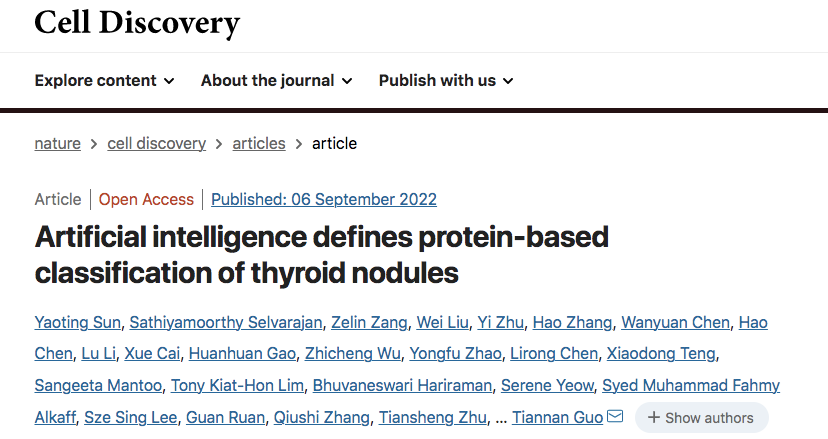
The latest paper published by Guo Tiannan's team, the picture is taken from Cell Discovery
In the latest study published by Tiannan Guo et al., researchers used pressure cycling technology to perform proteomic analysis on micro-tissue samples.
Based on 1724 paraffin-embedded (FFPE) thyroid tissues, the researchers found 19 protein biomarkers and built a model—a neural network model. The experimental results show that the accuracy of the model in judging benign and malignant thyroid nodules exceeds 91%.
To validate the model's performance, the researchers tested it on 288 samples from China, and its diagnostic results were 89 percent accurate. Another 294 samples collected from 12 clinical centers in Singapore and China showed an accuracy rate of 85% for the validation results.
In the molecular diagnosis of thyroid nodules, the auxiliary diagnosis method based on nucleic acid (DNA and RNA) is a relatively mature technology. However, RNA is easily degraded, which brings challenges to detection.
At the same time, the mutation used to distinguish benign from malignant nodules is still controversial, thus limiting its application.
In contrast, analysis at the molecular level of the protein is much more reliable. When a lesion occurs, the state of the lesion can be directly displayed at the level of protein molecules.
However, due to the complexity of proteome data, the workload of researchers will become very large. The addition of AI just solves this problem. Its computing speed and accuracy make the process of analyzing proteome data much easier for researchers. .
Professor Guo Tiannan also believes that if big health and AI can be combined, it will be the general trend of the times, whether from an academic perspective, from the perspective of the necessity of biological principles, or from the needs of the market and society.
References
1. Artificial intelligence defines protein-based classification of thyroid nodules. Cell.
2. Tiannan Guo|Artificial intelligence + proteomics: the underlying biological changes in drug research and development. Zhiyaobang.
https://mp.weixin.qq.com/s/HO3BCZix8vhhf1ZENv2Ndg
3. Proteomic and Metabolomic Characterization of COVID-19 Patient Sera.Cell.
https://www.cell.com/cell/fulltext/S0092-8674(20)30627-9
4. Multi-organ Proteomic Landscape of COVID-19 Autopsies. Cell.
https://www.cell.com/cell/fulltext/S0092-8674(21)00004-0
Comments