A completely self-supervised learning algorithm, recently published in the sub-journal of Nature, can track tiny changes in protein positioning based on images of proteins and predict how proteins work together in cells. This could be the key to further understanding many diseases and facilitating drug development. 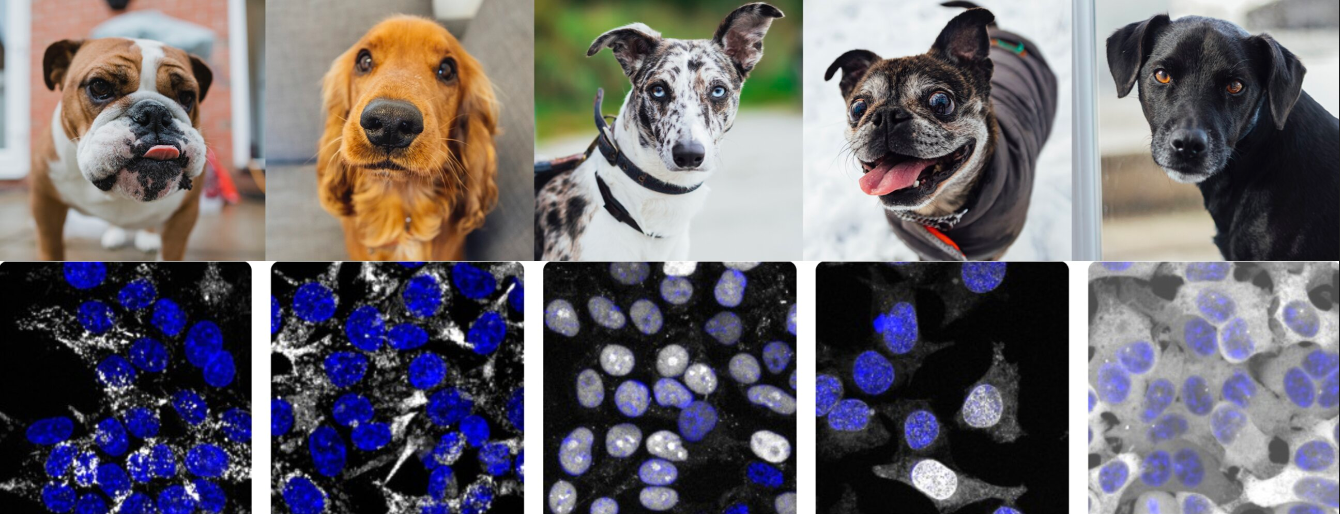
These proteins may appear in combination in different places in the cell, how exactly do they work together?
In late July, a research team from the Chan Zuckerberg Biohub (CZ Biohub) developed this fully self-supervised deep learning method called "Cytoself", which can be used without any prior knowledge. Microscopic images of proteins were quantitatively analyzed and compared.
The center was funded by Facebook founder Mark Zuckerberg and his wife Priscilla Chan.
How does it do it?
New algorithm quantifies and compares proteins in images
For decades, biologists have been trying various methods and tools to determine all possible positions and structures of proteins in cells to help us further understand how proteins work, and the emergence of Cytoself has quickly surfaced the answer to the question.
The research from the Chan-Zuckerberg Biocenter in the United States was published in Nature Methods on July 25. Titled "Self-supervised deep learning encodes high-resolution features of protein subcellular localization".
What makes Cytoself's algorithm different?
Simply put, it is able to identify the diversity and complexity of protein localization through self-supervised learning in machine learning.
Rather than feeding the algorithm individual examples one by one when training the Cytoself algorithm, the researchers opted for a self-supervised learning training scheme that revealed high-resolution maps of protein subcellular localization .
In a supervised learning model, humans must continuously teach the robot with a single example, that is, a large amount of knowledge about protein images needs to be input into the algorithm to achieve the training effect of the algorithm. This process is complicated and tedious for researchers. of. If the robot is limited to the limited number of examples that humans have trained it on, it may introduce certain biases into the system. Self-supervised learning can circumvent these shortcomings.
The researchers were amazed at the amount of information the Cytoself algorithm was trained to extract from protein images. Cytoself not only demonstrates the capabilities of machine learning algorithms, but also provides new perspectives for the study of cells and proteins.
"This is very exciting," said Loic Roye, the corresponding author of the paper. "We are applying artificial intelligence to a new kind of problem, and we are reproducing everything that humans know, and even discovering things that humans don't know yet."
Another corresponding author, Manuel D. Leonetti, said, "The machine converts each protein image into a mathematical vector, so researchers can compare images of proteins that look almost indistinguishable. We can also compare images of proteins to predict them . The way it works together in cells , it's kind of surprising."
Kobayashi, an expert in machine learning and high-speed imaging, said, "While there has been some previous work on protein images using self-supervised or unsupervised models, self-supervised learning has never been so successfully used to process such a large dataset, which The collection has more than 1 million images covering more than 1,300 proteins in human cells."
It is worth mentioning that these more than 1 million images come from the OpenCell database of CZ Biohub. The database aims to create a complete map of human cells, including the eventual description of the 20,000 or so proteins in cells.
It is reported that the next step of the research team will use Cytoself to track small changes in protein localization to identify different cellular states, such as normal cells and cancer cells, which may be the key to further understanding many diseases and promoting drug development.
In this regard, Kobayashi said that screening in the drug development process basically requires repeated trials, but with the Cytoself algorithm, scientists no longer need to experiment with thousands of proteins one by one. This method can effectively reduce costs and increase the speed of drug development.
What is the Chan Zuckerberg Biocenter?
Cytoself was invented by researchers at the Chan Zuckerberg Biological Center. What is the origin of this private research institution?
Officially launched in 2016, the Chan Zuckerberg Biocenter is headquartered in San Francisco and is a non-profit research center.
According to the China Science News, the center, co-founded by Facebook founder Mark Zukerberg and his wife Priscilla Chan, is located in San Francisco, California, USA. and non-profit research institutions that control various diseases. In early 2017, the center announced that it would grant unconditional funding to 47 researchers at three nearby research universities full of adventurous new ideas. The biocenter is also the first physical institution for "Operation Chan Zuckerberg" to enter the scientific field. At the beginning of its establishment in September 2016, Zuckerberg and the wife of a pediatrician jointly committed to the next 10 years. The center continues to invest $3 billion.
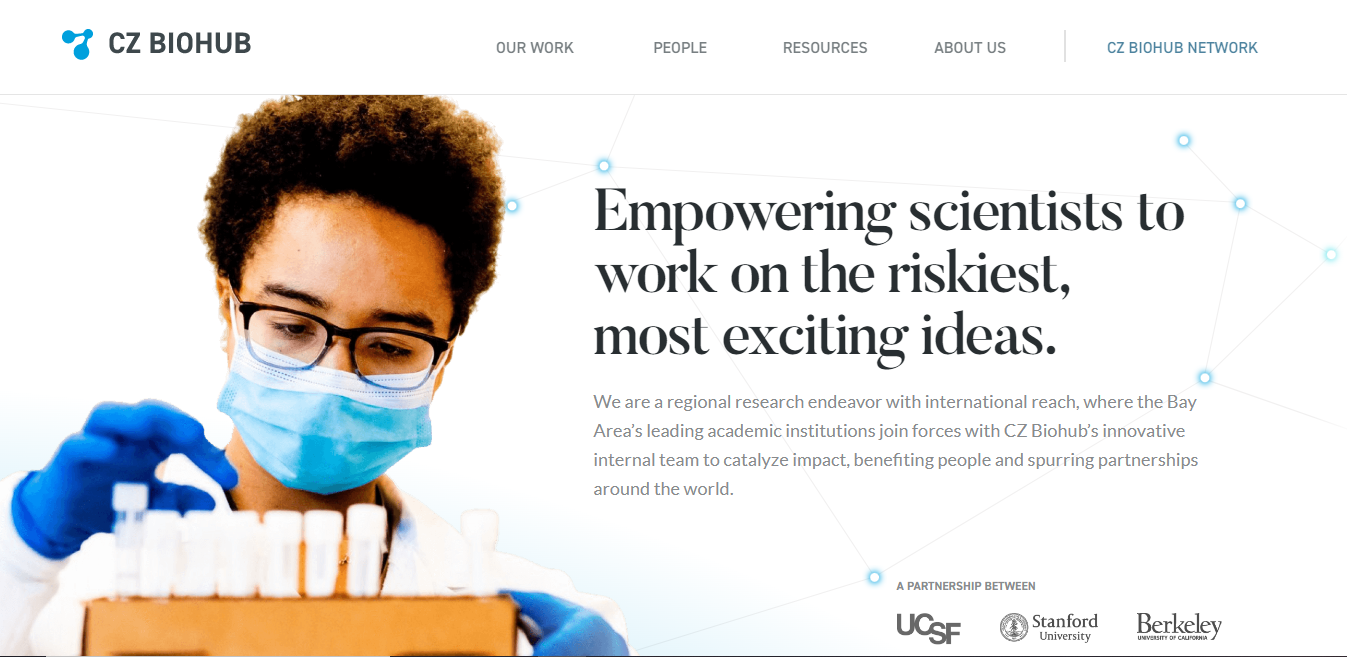
Dr. Joe DeRisi, co-president of the Chan Zuckerberg Biocenter, said, "The Chan Zuckerberg Biocenter will expand our global reach by creating new technology platforms, fundamental databases and large-scale cell biology pipelines. Pathogen detection work, more in-depth research in infectious diseases and basic science."
Artificial intelligence involved in life science research?
When it comes to Cytoself, we can't help but think of the famous AlphaFold. They both use machine learning algorithms to study proteins. But what's the difference between the two?
Developed by DeepMind, AlphaFold has predicted more than 200 million protein structures based on gene sequences.
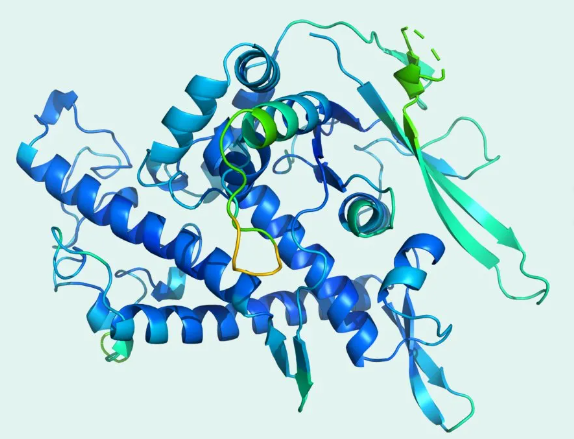
Overall, whether it is the AlphaFold system or the Cytoself algorithm, they are the results of the interdisciplinary integration of artificial intelligence (AI) and life science research. In the future, this interdisciplinary research method may be more and more, and it will also bring more surprises and discoveries to people.
References
1. Self-supervised deep learning encodes high-resolution features of protein subcellular localization.nature methods.
https://www.nature.com/articles/s41592-022-01541-z
2. AI can reveal new cell biology just by looking at images.phys.org.
https://phys.org/news/2022-08-ai-reveal-cell-biology-images.html
3. Home.czbiohub.
https://www.czbiohub.org/
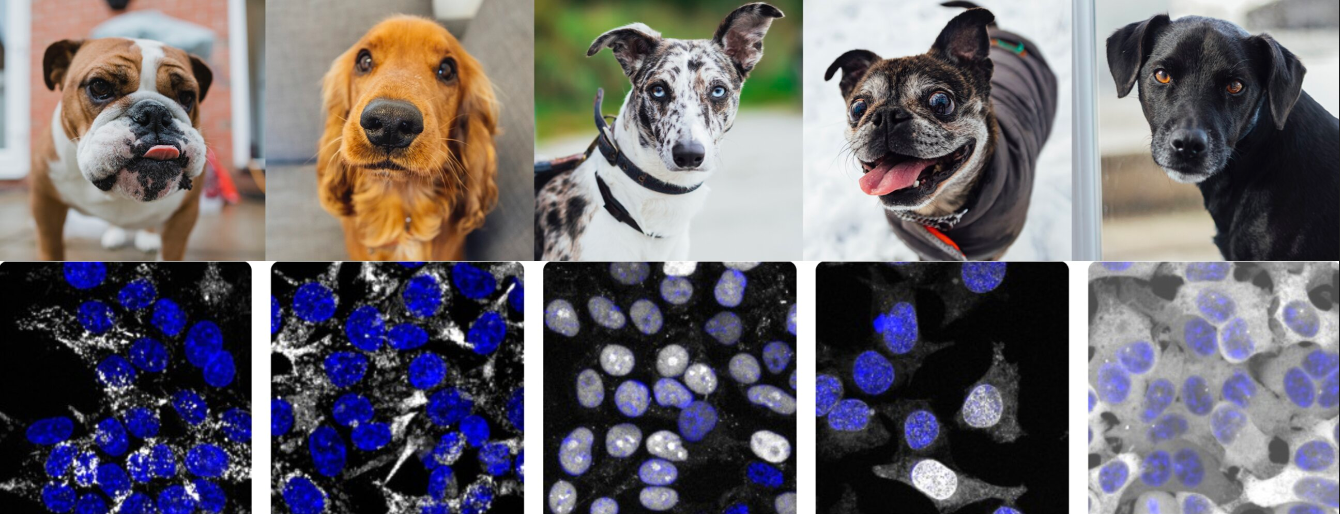
In addition to learning to identify and classify different dog breeds from images (top), a new machine learning method from CZ Biohub can distinguish different human proteins from fluorescence microscopy images (bottom). Credit: CZ Biohub
Each cell of the human body contains about 10,000 different types of proteins, which support almost all cellular activities and are called "little housekeepers" of various functions of the body. Some proteins work individually, while others work together to keep cells healthy.These proteins may appear in combination in different places in the cell, how exactly do they work together?
In late July, a research team from the Chan Zuckerberg Biohub (CZ Biohub) developed this fully self-supervised deep learning method called "Cytoself", which can be used without any prior knowledge. Microscopic images of proteins were quantitatively analyzed and compared.
The center was funded by Facebook founder Mark Zuckerberg and his wife Priscilla Chan.
How does it do it?
New algorithm quantifies and compares proteins in images
For decades, biologists have been trying various methods and tools to determine all possible positions and structures of proteins in cells to help us further understand how proteins work, and the emergence of Cytoself has quickly surfaced the answer to the question.
The research from the Chan-Zuckerberg Biocenter in the United States was published in Nature Methods on July 25. Titled "Self-supervised deep learning encodes high-resolution features of protein subcellular localization".
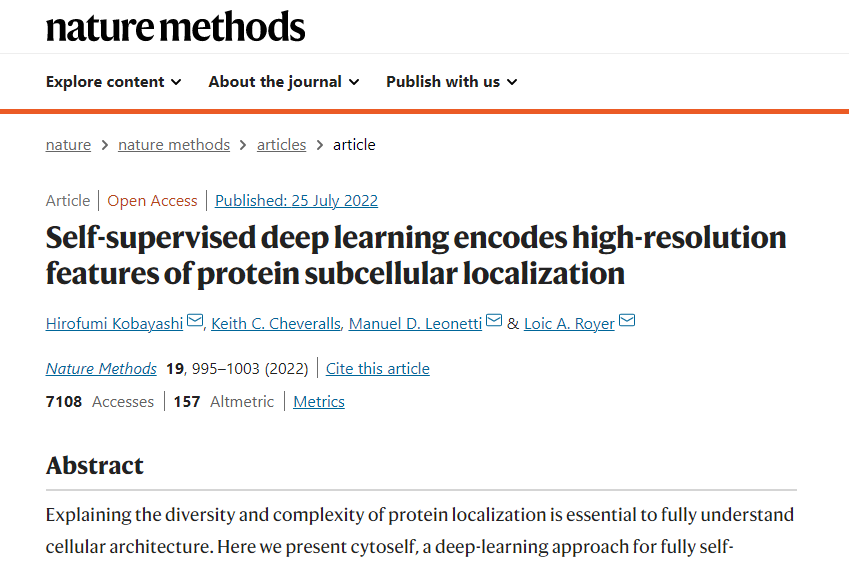
Simply put, it is able to identify the diversity and complexity of protein localization through self-supervised learning in machine learning.
Rather than feeding the algorithm individual examples one by one when training the Cytoself algorithm, the researchers opted for a self-supervised learning training scheme that revealed high-resolution maps of protein subcellular localization .
In a supervised learning model, humans must continuously teach the robot with a single example, that is, a large amount of knowledge about protein images needs to be input into the algorithm to achieve the training effect of the algorithm. This process is complicated and tedious for researchers. of. If the robot is limited to the limited number of examples that humans have trained it on, it may introduce certain biases into the system. Self-supervised learning can circumvent these shortcomings.
The researchers were amazed at the amount of information the Cytoself algorithm was trained to extract from protein images. Cytoself not only demonstrates the capabilities of machine learning algorithms, but also provides new perspectives for the study of cells and proteins.
"This is very exciting," said Loic Roye, the corresponding author of the paper. "We are applying artificial intelligence to a new kind of problem, and we are reproducing everything that humans know, and even discovering things that humans don't know yet."
Another corresponding author, Manuel D. Leonetti, said, "The machine converts each protein image into a mathematical vector, so researchers can compare images of proteins that look almost indistinguishable. We can also compare images of proteins to predict them . The way it works together in cells , it's kind of surprising."
Kobayashi, an expert in machine learning and high-speed imaging, said, "While there has been some previous work on protein images using self-supervised or unsupervised models, self-supervised learning has never been so successfully used to process such a large dataset, which The collection has more than 1 million images covering more than 1,300 proteins in human cells."
It is worth mentioning that these more than 1 million images come from the OpenCell database of CZ Biohub. The database aims to create a complete map of human cells, including the eventual description of the 20,000 or so proteins in cells.
It is reported that the next step of the research team will use Cytoself to track small changes in protein localization to identify different cellular states, such as normal cells and cancer cells, which may be the key to further understanding many diseases and promoting drug development.
In this regard, Kobayashi said that screening in the drug development process basically requires repeated trials, but with the Cytoself algorithm, scientists no longer need to experiment with thousands of proteins one by one. This method can effectively reduce costs and increase the speed of drug development.
What is the Chan Zuckerberg Biocenter?
Cytoself was invented by researchers at the Chan Zuckerberg Biological Center. What is the origin of this private research institution?
Officially launched in 2016, the Chan Zuckerberg Biocenter is headquartered in San Francisco and is a non-profit research center.
According to the China Science News, the center, co-founded by Facebook founder Mark Zukerberg and his wife Priscilla Chan, is located in San Francisco, California, USA. and non-profit research institutions that control various diseases. In early 2017, the center announced that it would grant unconditional funding to 47 researchers at three nearby research universities full of adventurous new ideas. The biocenter is also the first physical institution for "Operation Chan Zuckerberg" to enter the scientific field. At the beginning of its establishment in September 2016, Zuckerberg and the wife of a pediatrician jointly committed to the next 10 years. The center continues to invest $3 billion.
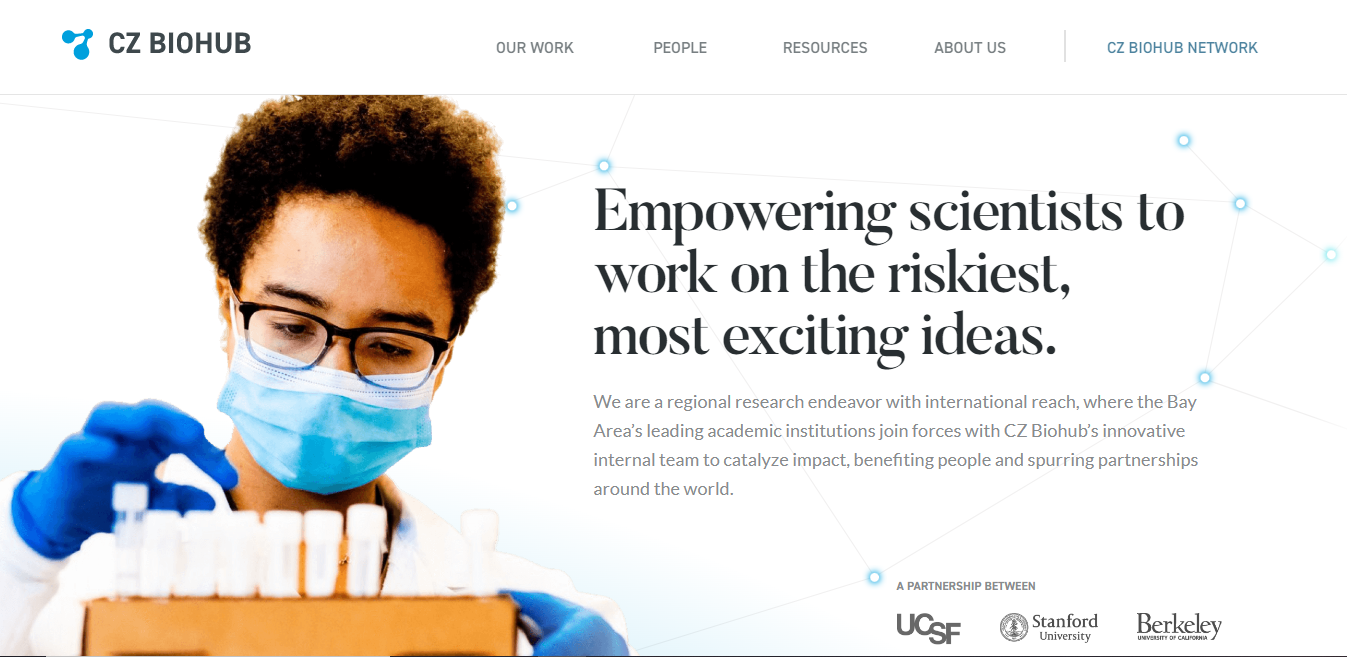
CZ Biohub Homepage
Simply put, the Chan Zuckerberg Biocenter supports rigorous, quantitative research in cell biology to combat diseases caused by cellular dysregulation. They also help humanity respond to threats from existing and emerging pathogens. In addition to this, the Chan Zuckerberg Biocenter open sourced their tools and techniques to fellow researchers.Dr. Joe DeRisi, co-president of the Chan Zuckerberg Biocenter, said, "The Chan Zuckerberg Biocenter will expand our global reach by creating new technology platforms, fundamental databases and large-scale cell biology pipelines. Pathogen detection work, more in-depth research in infectious diseases and basic science."
Artificial intelligence involved in life science research?
When it comes to Cytoself, we can't help but think of the famous AlphaFold. They both use machine learning algorithms to study proteins. But what's the difference between the two?
Developed by DeepMind, AlphaFold has predicted more than 200 million protein structures based on gene sequences.
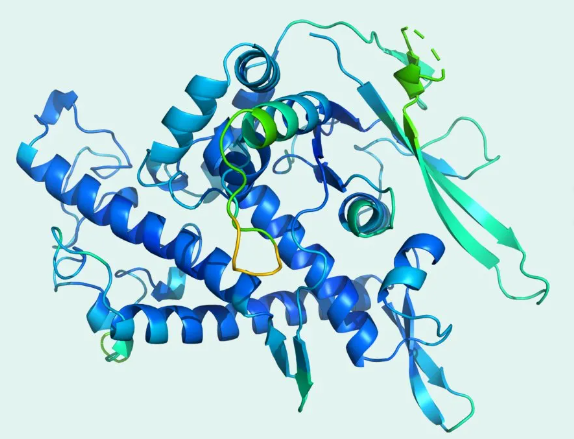
Protein structure predicted by AlphaFold. Figure: DeepMind
Overall, whether it is the AlphaFold system or the Cytoself algorithm, they are the results of the interdisciplinary integration of artificial intelligence (AI) and life science research. In the future, this interdisciplinary research method may be more and more, and it will also bring more surprises and discoveries to people.
References
1. Self-supervised deep learning encodes high-resolution features of protein subcellular localization.nature methods.
https://www.nature.com/articles/s41592-022-01541-z
2. AI can reveal new cell biology just by looking at images.phys.org.
https://phys.org/news/2022-08-ai-reveal-cell-biology-images.html
3. Home.czbiohub.
https://www.czbiohub.org/
Comments