Does AI also have human-like "auditory cortex" and "prefrontal cortex" mechanisms?
Recently, researchers from Meta AI, Columbia University, University of Toronto, etc. have completed a study on the similarity between deep learning models and the human brain.
"Simulating human intelligence is a distant goal. Nonetheless, the emergence of brain-like functions in self-supervised algorithms suggests we may be on the right track," tweeted one of the paper's authors, a researcher at Meta AI.
So what did they find?
The study found that the AI model Wav2Vec 2.0 is very similar to the way the human brain processes speech, and even AI, like humans, has a stronger ability to discriminate "native languages". For example, the French model is more likely to perceive stimuli from French than the English model.
In a demo video posted by Jean-Rémi King on Twitter, he shows how the AI model corresponds to the human brain: the auditory cortex best matches the Transformer's first layer (blue), while the prefrontal cortex matches the Transformer's deepest layer. Layer (red) fits best.
Wav2Vec 2.0 received 600 hours of phonological training, which is roughly equivalent to what newborns are exposed to in the early stages of language acquisition. The researchers compared this model with the brain activity of 412 volunteers (351 English speakers, 28 French speakers and 33 Mandarin speakers).
Scientists had participants listen to an audiobook in their native language for an hour and then took functional magnetic resonance imaging (fMRI) recordings of their brains. The researchers compared these brain activities to each layer of the Wav2Vec 2.0 model and several variants, including a random (untrained) Wav2Vec 2.0 model, a model trained on 600 hours of non-speech, and a model trained on 600 hours of non-native speech. Models trained on 600 hours of native language training, and models trained directly on speech-to-text in the participant's native language.
The experiment has four important findings.
First, Wav2Vec 2.0 uses self-supervised learning to acquire speech waveforms similar to what is seen in the human brain. Second, the functional hierarchy of the Transformer layer matches the cortical hierarchy of speech in the brain, revealing the whole-brain arrangement of speech processing in unprecedented detail. Third, the model's representations of hearing, speech, and language converge with those of the human brain. Fourth, behavioral comparisons of the model with speech discrimination exercises performed by another 386 human participants indicated a common language specialization.
These experimental results demonstrate that 600 hours of self-supervised learning is sufficient to produce a model that is functionally equivalent to human speech perception. The material required for Wav2Vec 2.0 to learn language-specific representations is comparable to the amount of "data" that infants are exposed to during the process of learning to speak.
Yann LeCun, one of the "Big Three" of deep learning, hailed it as "excellent work", and the team's research showed that there is indeed a strong correlation between the hierarchical activity of Transformers trained in self-supervised learning in speech and the activity of the human auditory cortex.
Jesse Engel, a researcher at Google Brain, said the study takes visualization filters to the next level. Now, not only can you see what they look like in "pixel space", but what they look like in "brain-like space" can also be simulated.
But some critics, such as Patrick Mineault, a postdoctoral fellow in neuroscience at the University of California, Los Angeles, are somewhat skeptical that the study actually measures speech processing in the human brain. Because fMRI measures signals very slowly compared to the speed of human speech, that means great care needs to be taken when interpreting the results. Mineault also said he didn't think the study was unreliable, but the study needed to give some more convincing data.
Meta AI has actually been looking for connections between AI algorithms and the human brain. Meta AI previously announced that they will be working with neuroimaging centers Neurospin (CEA) and INRIA to try to decode how the human brain and deep learning algorithms trained on language tasks respond to the same piece of text.
For example, by comparing human brain scans with deep learning algorithms when a person is actively reading, speaking, or listening, and given the same set of words and sentences to decipher, researchers hope to find out about brain biology and artificial neural The similarities between the networks, along with key structural and behavioral differences, help explain why humans process language so much more efficiently than machines.
"What we're doing is trying to compare brain activity with machine learning algorithms to understand how the brain works and try to improve machine learning," said Meta AI research scientist Jean-Rémi King.
Recently, researchers from Meta AI, Columbia University, University of Toronto, etc. have completed a study on the similarity between deep learning models and the human brain.
"Simulating human intelligence is a distant goal. Nonetheless, the emergence of brain-like functions in self-supervised algorithms suggests we may be on the right track," tweeted one of the paper's authors, a researcher at Meta AI.
So what did they find?
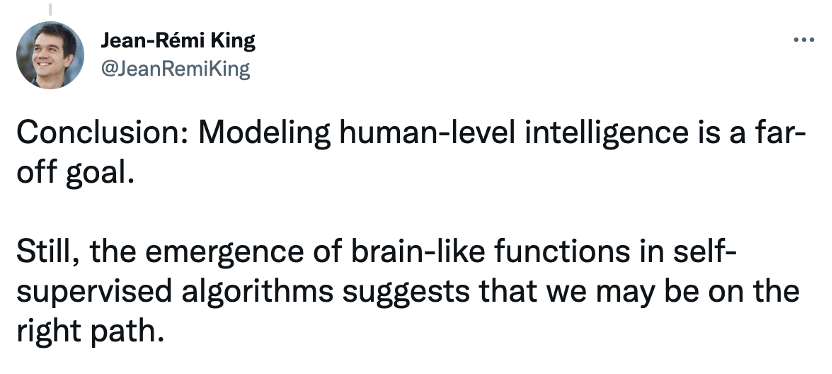
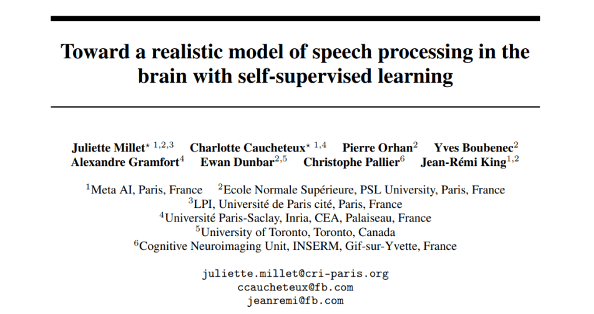
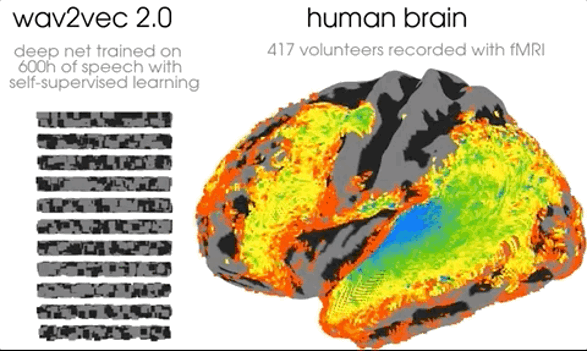
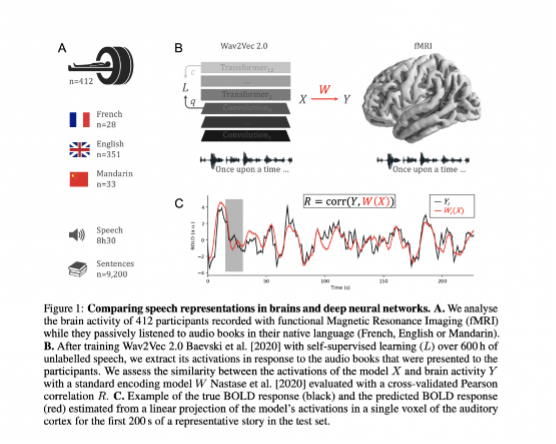
The experiment has four important findings.
First, Wav2Vec 2.0 uses self-supervised learning to acquire speech waveforms similar to what is seen in the human brain. Second, the functional hierarchy of the Transformer layer matches the cortical hierarchy of speech in the brain, revealing the whole-brain arrangement of speech processing in unprecedented detail. Third, the model's representations of hearing, speech, and language converge with those of the human brain. Fourth, behavioral comparisons of the model with speech discrimination exercises performed by another 386 human participants indicated a common language specialization.
These experimental results demonstrate that 600 hours of self-supervised learning is sufficient to produce a model that is functionally equivalent to human speech perception. The material required for Wav2Vec 2.0 to learn language-specific representations is comparable to the amount of "data" that infants are exposed to during the process of learning to speak.
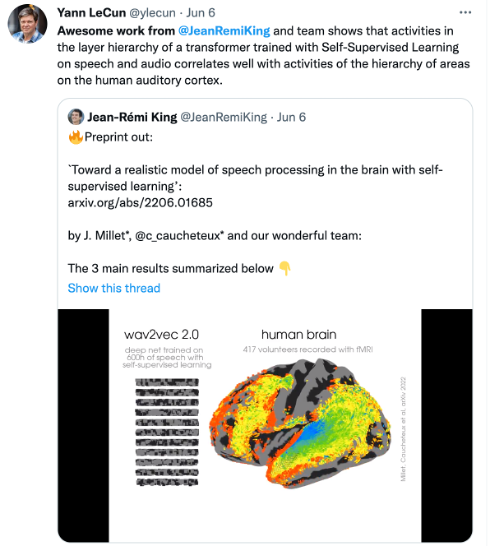
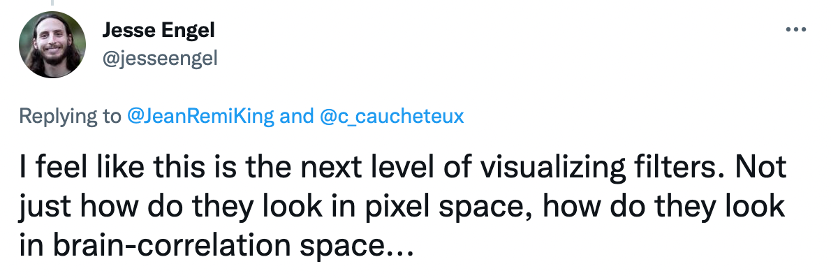
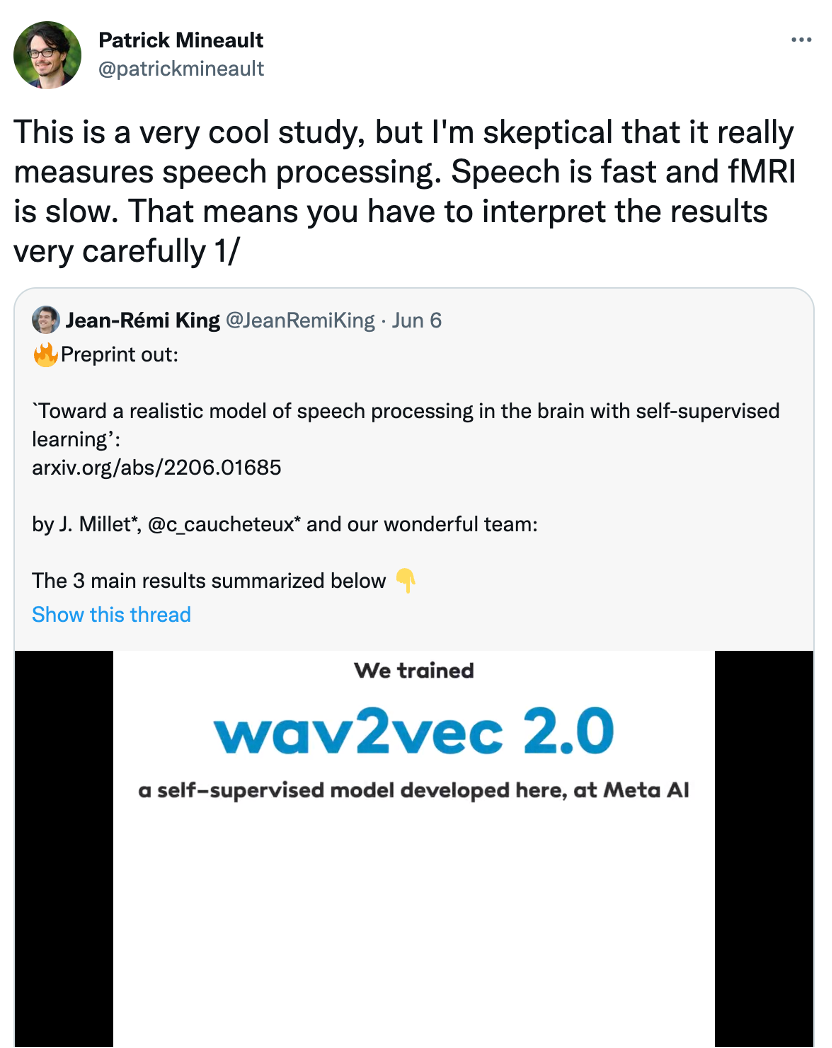
Meta AI has actually been looking for connections between AI algorithms and the human brain. Meta AI previously announced that they will be working with neuroimaging centers Neurospin (CEA) and INRIA to try to decode how the human brain and deep learning algorithms trained on language tasks respond to the same piece of text.
For example, by comparing human brain scans with deep learning algorithms when a person is actively reading, speaking, or listening, and given the same set of words and sentences to decipher, researchers hope to find out about brain biology and artificial neural The similarities between the networks, along with key structural and behavioral differences, help explain why humans process language so much more efficiently than machines.
"What we're doing is trying to compare brain activity with machine learning algorithms to understand how the brain works and try to improve machine learning," said Meta AI research scientist Jean-Rémi King.
Comments