"This year is a critical year for the implementation of large models. After the exploration period and breakthrough period in previous years, large models have reached the promotion period to a certain extent. Then we will face how to implement them and how to use them in real application scenarios. The problem of generating value. From the perspective of application landing, for the landing of large models, the most critical problem to be solved is the gap between this cutting-edge technology and real application scenarios. How can it fully match the requirements of the application landing? This is The core problem to be solved by large models this year.” Recently, Wu Tian, Vice President of Baidu Group and Deputy Director of the National Engineering Research Center for Deep Learning Technology and Application, said at the WAVE SUMMIT 2022 Deep Learning Developer Summit.
So how to solve it, how to move forward? Wu Tian specifically summarized it into three points.
The first is to build a large model system, and this system can be connected with application scenarios. The second is supporting platforms and tools, lowering the application threshold, and supporting the entire landing application in the whole process and end-to-end. The third is the need for ecological support, including the construction of application ecology and hardware ecology.
At the WAVE SUMMIT Deep Learning Developer Summit in the spring of 2022, Baidu released ten large models, the first industry large model, and a series of tools and platforms, including large model development kits, large model APIs, and built-in large model capabilities. EasyDL and BML development platform, as well as Wenxin·Yanggu community. It's easy to feel dazzled if you don't understand the logic, but you can understand all of Baidu's actions in this regard by understanding the above three points.
The big model of the first industry: do not seek "all-in-one", and each layered system performs its own duties
"In the stage of large-scale production of AI industry, the versatility of deep learning technology is becoming stronger and stronger, the standardization, automation and modularization of deep learning platforms are becoming more and more prominent, and the application of deep learning is becoming more and more extensive and in-depth, and has blossomed everywhere. Pre-training The rise of large models has further enhanced the versatility of artificial intelligence. Large models have the characteristics of good effect, strong generalization, and high degree of standardization of R&D processes, and are becoming a new base for artificial intelligence technology and applications.” Baidu Chief Technology Officer, Wang Haifeng, director of the National Engineering Research Center for Deep Learning Technology and Applications, said.
As early as at the WAVE SUMMIT summit in May 2021, Wu Tian talked about the three stages of enterprise AI application: one is the "pioneer's path-finding stage", where a small number of pioneers introduce new technologies into the enterprise for exploration and prototype verification; Workshop application stage", some companies gradually set up small teams and introduce technology; the third is the "industrial large-scale production stage", in which large-scale human resources and other resources within the company are coordinated to carry out artificial intelligence research and development.
Then in this stage of AI industrial production, Baidu's idea is not to build a large model to "take all" all problems, but to build a layered system. The Flying Paddle Wenxin model contains three types of models: basic model, task model and industry model. This time, 10 large models including ERNIE 3.0 Zeus (Zeus), the first 100 billion large model with open API calls in China, belong to these three categories.
The basic large model has the characteristics of learning data, large amount of knowledge, large scale of parameters, and the highest versatility. However, the direct use of the basic model often has a certain gap with the demanding application requirements of the scene. Therefore, on the basis of the general model, Baidu has added two types of models: the task model and the industry model.
The task model is mainly oriented to specific tasks, such as information extraction, dialogue, and search in the NLP field, as well as commodity image and text search in the visual field, and document image understanding.
The industry model is based on the general Wenxin model, which mines industry field data from massive and extensive data, and cooperates with leading companies or institutions in the industry to introduce industry-specific data and knowledge. "The main purpose is to combine and learn the general basic model and industry-level knowledge. The key point of the industry model is to introduce the unique knowledge and data in the industry, as well as the knowledge and know-how of in-depth industry experts. Experts, design corresponding pre-training tasks for the industry together. In this way, the general model will truly become a model that is more applicable to the industry." Wu Tian told The Paper (www.thepaper.cn).
Wu Tian introduced that in the fields of energy, power and finance, Wenxin and State Grid have developed a knowledge-enhanced NLP model for the energy industry "State Grid-Baidu·Wenxin", and Shanghai Pudong Development Bank has developed a knowledge-enhanced NLP model for the financial industry. Pudong Development-Baidu·Wenxin".
The value behind such cooperation can be understood from the speeches of both parties.
Taking the energy and power industry as an example, Wu Tian believes that it is more important to promote the large model of the industry to work with experts from the State Grid to introduce the sample data and unique knowledge accumulated in the power business, and in the training, combine the pre-training algorithms and power Experience in domain business and algorithms, design algorithms such as entity discrimination in the electric power domain and document discrimination in the electric power domain as pre-training tasks, so that the Wenxin model can deeply learn electric power expertise.
Dr. Jiang Wei, head of the artificial intelligence work of the Digital Work Department of State Grid Corporation of China, said that as the vanguard of the digital transformation of central enterprises, State Grid Corporation of China and Baidu have jointly built industry-level artificial intelligence infrastructure, and explored and developed a joint large-scale model of electric power artificial intelligence. It not only improves the accuracy of traditional power-specific models, but also greatly reduces the research and development threshold, and realizes the overall optimization of computing power, data, technology and other resources. In the next step, State Grid Corporation of China will continue to deepen the technical cooperation between the two parties, promote the technical research and application exploration of artificial intelligence large models in the field of electricity, and build artificial intelligence large models with more power characteristics for more typical power business scenarios.
Similarly, the SPD-Baidu·Wenxin large model conducts industry data mining based on Wenxin. Combined with the industry data and knowledge accumulated in SPD's scenarios, the technical and business experts of both parties can cooperate to design targeted financial report field discrimination, financial customer service Q&A matching and other predictions. training tasks.
In addition to the industry model, a total of eight Wenxin basic models and task models have been released this time, including: ERNIE 3.0 Zeus, a 100 billion model that integrates task-related knowledge, VIMER-UFO 2.0 for multi-task visual representation learning, and commodity map Text Search Representation Learning VIMER-UMS, Document Image Representation Learning VIMER-StrucTexT 2.0, Speech-Language Cross-modal Model ERNIE-SAT, Geography-Language Cross-modal Large Model ERNIE-GeoL, and Compound Representation Learning for Biocomputing HELIX-GEM and protein structure analysis HELIX-Fold.
"A good horse with a good saddle": companion tools and platforms for large models
In order to give full play to the value of large models in application scenarios and lower the threshold for use, Baidu has built supporting tools and platforms.
The large model suite mainly provides four capabilities, such as a variety of data preprocessing tools that help developers reduce data preparation costs; at the same time, considering that large models need to be combined with scene problem transfer learning, Baidu provides a variety of fine-tuning tools, including adversarial learning. , small sample learning and other fine-tuning methods, as well as new large model fine-tuning tools such as Prompt-tuning. In view of the high cost of large model deployment, Wenxin large model tools and platforms are equipped with high-performance deployment solutions for model miniaturization, including performance acceleration solutions, and more than 60 basic tasks of NLP and CV are preset at the same time.
The Wenxin large model and related tools can be used in the EasyDL and BML platforms of Flying Paddle Enterprise Edition. According to Baidu, more than 10,000 users on the platform have used pre-trained large models, created more than 30,000 tasks, and applied them to a large number of scenarios such as power transmission inspection, parts defect detection, agricultural pest and disease identification, and news information creation. middle. On the platform, through the development of AI application models through the large model mechanism, the amount of data annotation is reduced by an average of 70%, and the effect is increased by an average of 10.7%. The Wenxin model also provides a direct API call method. ERNIE 3.0 Zeus, PLATO, and ERNIE-ViLG can all be accessed and called directly by the user through the API.
In general, the core features of Fei Pao Wenxin's large model have two points: industrial level and knowledge enhancement.
"Industrial level" means that Wenxin's entire technology is polished in the actual industrial application process. For example, how to design the labeling of data, how much data is recommended, the corresponding transfer learning method, etc. These supporting tools and platforms, including the newly released large model API, large model development kit, platform portal, etc., are all improving the feasibility of real applications.
"Knowledge enhancement" means that compared with other industry models, Baidu integrates data and knowledge through the introduction of knowledge graphs, with the goal of making Wenxin model more efficient and interpretable. By improving the generality and generalization of large models, development difficulty can be reduced and less labeled data can be obtained.
On the whole, whether it is a flying paddle platform or the Wenxin large model specific to the flying paddle model library, the concept behind it is to lower the threshold of AI use, improve the technical versatility, and strengthen the standardization, automation and modularization of technology and platforms. .
Wu Tian believes that open source and openness are also very direct ways to lower the threshold. Because the application of AI is not only a technical issue, but more importantly, it is combined with industries and scenarios. And through open source, the ability of group intelligence innovation and deep collaboration can also be significantly improved, which can accelerate the intelligent transformation of enterprises. "Among the 10 large models released today, 7 models are open source, and open source is what Wenxin's large model has been doing." Wu Tian told The Paper (www.thepaper.cn).
How to tackle the training and inference challenges of large models?
"As deep learning technicians, we clearly recognize that AI large model is a new breakthrough in deep learning technology, which further enhances the versatility of AI technology and brings a new AI research and development paradigm. For the majority of developers, Based on the pre-trained large model, it is possible to develop a better AI model for the scene at a lower cost and with a low threshold." Wu Tian said.
The training and reasoning of Wenxin's large model rely on the support of the deep learning platform. At the same time, the large model, as an important member of the industrial-level model library in the Flying Paddle platform, has become an indispensable ability for the Flying Paddle platform to support AI innovation.
The challenges of large model training mainly come from "big", the large scale of model parameters, and the differences in the characteristics of different models and computing power platforms, which bring practical challenges to large model training. The distributed architecture of Flying Paddle takes these differences into consideration as a whole, uses an end-to-end adaptive distributed architecture, and automatically selects parallel strategies, automatically optimizes, and executes efficiently according to the characteristics of the model and computing power platform. Efficiency is also taken into account. Its innovation in parallel training strategy is to support adaptive parallel training for heterogeneous hardware, create a large model training solution that combines framework, computing power and algorithm, and achieve end-to-end extreme performance optimization.
Compared with training, large model inference faces greater challenges. Efficient reasoning of large models is the key to the realization of industrial applications of large models. At the deployment level of large models, Fei Pao has launched a full-process deployment plan for compression, reasoning, and service of large models to help large models land better.
It first makes the model lightweight through precision lossless model compression technology, and then fully mobilizes computing resources through adaptive distributed reasoning technology. Finally, through large-scale service deployment, the large model can be truly applied. The overall solution is universal and extensible, and can widely support different types of model structures to achieve high-speed reasoning. Currently, it has supported real-time online applications of large models such as natural language understanding, dialogue, and cross-modal generation.
These efforts are all to make the large model closer to the industry and land in the industry, not just laboratory technology.
Up to now, the Wenxin model has been used in industries such as industry, energy, education, finance, communications, and media, such as parts quality inspection in the industrial field, transmission line inspection in the energy field, inspiration for writing in the education industry, and financial industry. Contract information extraction, etc., really help enterprises reduce costs and increase efficiency and stimulate innovation. At the same time, the Wenxin model is also fully applied to Internet products such as intelligent search, information flow, and smart speakers to improve the efficiency and effect of users' acquisition of information, knowledge and services.
In general, Wu Tian gave three key paths to support the landing of Fei Pao Wenxin's large-scale model industry: build a large-scale model system that is more suitable for the needs of the scene, provide tools and methods to support the application landing in the whole process, and build an openness that stimulates innovation. ecology. Part of this ecological construction is the Wenxin·Yangu community, whose goal is to allow more people to have zero-distance access to AI large-scale model technology and stimulate innovation and creativity.
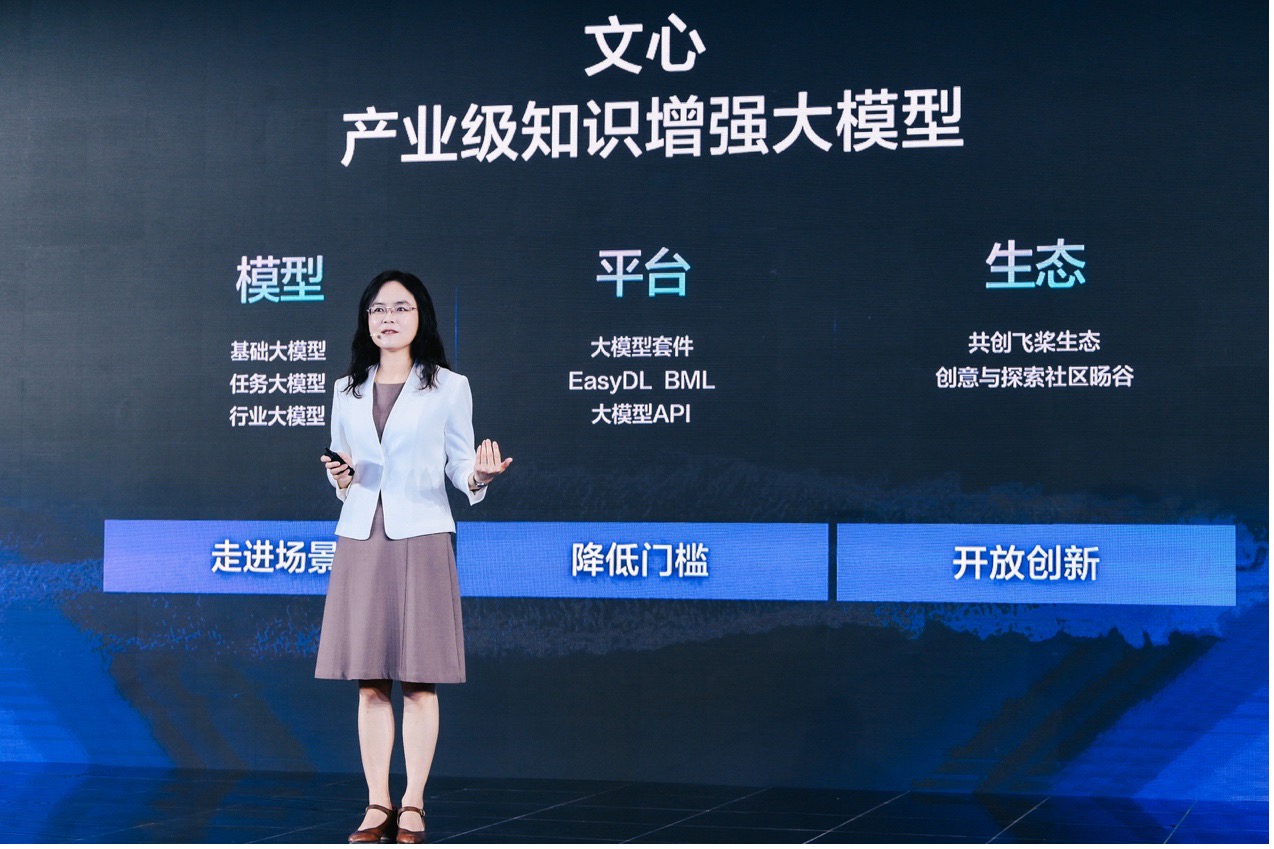
The first is to build a large model system, and this system can be connected with application scenarios. The second is supporting platforms and tools, lowering the application threshold, and supporting the entire landing application in the whole process and end-to-end. The third is the need for ecological support, including the construction of application ecology and hardware ecology.
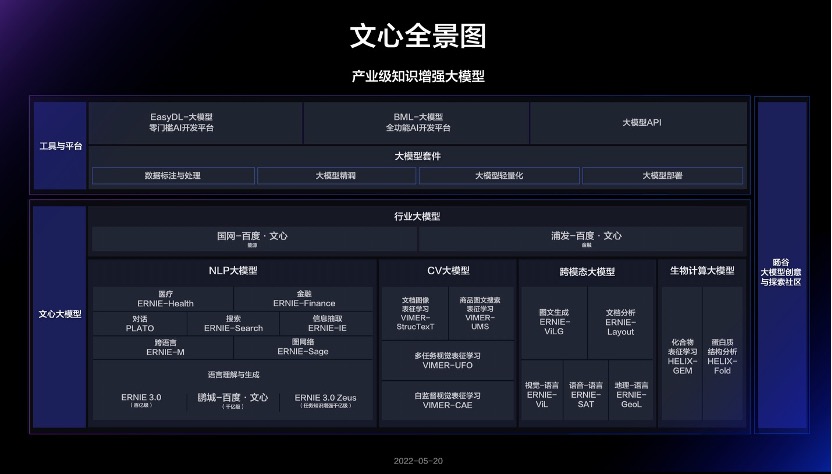
The big model of the first industry: do not seek "all-in-one", and each layered system performs its own duties
"In the stage of large-scale production of AI industry, the versatility of deep learning technology is becoming stronger and stronger, the standardization, automation and modularization of deep learning platforms are becoming more and more prominent, and the application of deep learning is becoming more and more extensive and in-depth, and has blossomed everywhere. Pre-training The rise of large models has further enhanced the versatility of artificial intelligence. Large models have the characteristics of good effect, strong generalization, and high degree of standardization of R&D processes, and are becoming a new base for artificial intelligence technology and applications.” Baidu Chief Technology Officer, Wang Haifeng, director of the National Engineering Research Center for Deep Learning Technology and Applications, said.
As early as at the WAVE SUMMIT summit in May 2021, Wu Tian talked about the three stages of enterprise AI application: one is the "pioneer's path-finding stage", where a small number of pioneers introduce new technologies into the enterprise for exploration and prototype verification; Workshop application stage", some companies gradually set up small teams and introduce technology; the third is the "industrial large-scale production stage", in which large-scale human resources and other resources within the company are coordinated to carry out artificial intelligence research and development.
Then in this stage of AI industrial production, Baidu's idea is not to build a large model to "take all" all problems, but to build a layered system. The Flying Paddle Wenxin model contains three types of models: basic model, task model and industry model. This time, 10 large models including ERNIE 3.0 Zeus (Zeus), the first 100 billion large model with open API calls in China, belong to these three categories.
The basic large model has the characteristics of learning data, large amount of knowledge, large scale of parameters, and the highest versatility. However, the direct use of the basic model often has a certain gap with the demanding application requirements of the scene. Therefore, on the basis of the general model, Baidu has added two types of models: the task model and the industry model.
The task model is mainly oriented to specific tasks, such as information extraction, dialogue, and search in the NLP field, as well as commodity image and text search in the visual field, and document image understanding.
The industry model is based on the general Wenxin model, which mines industry field data from massive and extensive data, and cooperates with leading companies or institutions in the industry to introduce industry-specific data and knowledge. "The main purpose is to combine and learn the general basic model and industry-level knowledge. The key point of the industry model is to introduce the unique knowledge and data in the industry, as well as the knowledge and know-how of in-depth industry experts. Experts, design corresponding pre-training tasks for the industry together. In this way, the general model will truly become a model that is more applicable to the industry." Wu Tian told The Paper (www.thepaper.cn).
Wu Tian introduced that in the fields of energy, power and finance, Wenxin and State Grid have developed a knowledge-enhanced NLP model for the energy industry "State Grid-Baidu·Wenxin", and Shanghai Pudong Development Bank has developed a knowledge-enhanced NLP model for the financial industry. Pudong Development-Baidu·Wenxin".
The value behind such cooperation can be understood from the speeches of both parties.
Taking the energy and power industry as an example, Wu Tian believes that it is more important to promote the large model of the industry to work with experts from the State Grid to introduce the sample data and unique knowledge accumulated in the power business, and in the training, combine the pre-training algorithms and power Experience in domain business and algorithms, design algorithms such as entity discrimination in the electric power domain and document discrimination in the electric power domain as pre-training tasks, so that the Wenxin model can deeply learn electric power expertise.
Dr. Jiang Wei, head of the artificial intelligence work of the Digital Work Department of State Grid Corporation of China, said that as the vanguard of the digital transformation of central enterprises, State Grid Corporation of China and Baidu have jointly built industry-level artificial intelligence infrastructure, and explored and developed a joint large-scale model of electric power artificial intelligence. It not only improves the accuracy of traditional power-specific models, but also greatly reduces the research and development threshold, and realizes the overall optimization of computing power, data, technology and other resources. In the next step, State Grid Corporation of China will continue to deepen the technical cooperation between the two parties, promote the technical research and application exploration of artificial intelligence large models in the field of electricity, and build artificial intelligence large models with more power characteristics for more typical power business scenarios.
Similarly, the SPD-Baidu·Wenxin large model conducts industry data mining based on Wenxin. Combined with the industry data and knowledge accumulated in SPD's scenarios, the technical and business experts of both parties can cooperate to design targeted financial report field discrimination, financial customer service Q&A matching and other predictions. training tasks.
In addition to the industry model, a total of eight Wenxin basic models and task models have been released this time, including: ERNIE 3.0 Zeus, a 100 billion model that integrates task-related knowledge, VIMER-UFO 2.0 for multi-task visual representation learning, and commodity map Text Search Representation Learning VIMER-UMS, Document Image Representation Learning VIMER-StrucTexT 2.0, Speech-Language Cross-modal Model ERNIE-SAT, Geography-Language Cross-modal Large Model ERNIE-GeoL, and Compound Representation Learning for Biocomputing HELIX-GEM and protein structure analysis HELIX-Fold.
"A good horse with a good saddle": companion tools and platforms for large models
In order to give full play to the value of large models in application scenarios and lower the threshold for use, Baidu has built supporting tools and platforms.
The large model suite mainly provides four capabilities, such as a variety of data preprocessing tools that help developers reduce data preparation costs; at the same time, considering that large models need to be combined with scene problem transfer learning, Baidu provides a variety of fine-tuning tools, including adversarial learning. , small sample learning and other fine-tuning methods, as well as new large model fine-tuning tools such as Prompt-tuning. In view of the high cost of large model deployment, Wenxin large model tools and platforms are equipped with high-performance deployment solutions for model miniaturization, including performance acceleration solutions, and more than 60 basic tasks of NLP and CV are preset at the same time.
The Wenxin large model and related tools can be used in the EasyDL and BML platforms of Flying Paddle Enterprise Edition. According to Baidu, more than 10,000 users on the platform have used pre-trained large models, created more than 30,000 tasks, and applied them to a large number of scenarios such as power transmission inspection, parts defect detection, agricultural pest and disease identification, and news information creation. middle. On the platform, through the development of AI application models through the large model mechanism, the amount of data annotation is reduced by an average of 70%, and the effect is increased by an average of 10.7%. The Wenxin model also provides a direct API call method. ERNIE 3.0 Zeus, PLATO, and ERNIE-ViLG can all be accessed and called directly by the user through the API.
In general, the core features of Fei Pao Wenxin's large model have two points: industrial level and knowledge enhancement.
"Industrial level" means that Wenxin's entire technology is polished in the actual industrial application process. For example, how to design the labeling of data, how much data is recommended, the corresponding transfer learning method, etc. These supporting tools and platforms, including the newly released large model API, large model development kit, platform portal, etc., are all improving the feasibility of real applications.
"Knowledge enhancement" means that compared with other industry models, Baidu integrates data and knowledge through the introduction of knowledge graphs, with the goal of making Wenxin model more efficient and interpretable. By improving the generality and generalization of large models, development difficulty can be reduced and less labeled data can be obtained.
On the whole, whether it is a flying paddle platform or the Wenxin large model specific to the flying paddle model library, the concept behind it is to lower the threshold of AI use, improve the technical versatility, and strengthen the standardization, automation and modularization of technology and platforms. .
Wu Tian believes that open source and openness are also very direct ways to lower the threshold. Because the application of AI is not only a technical issue, but more importantly, it is combined with industries and scenarios. And through open source, the ability of group intelligence innovation and deep collaboration can also be significantly improved, which can accelerate the intelligent transformation of enterprises. "Among the 10 large models released today, 7 models are open source, and open source is what Wenxin's large model has been doing." Wu Tian told The Paper (www.thepaper.cn).
How to tackle the training and inference challenges of large models?
"As deep learning technicians, we clearly recognize that AI large model is a new breakthrough in deep learning technology, which further enhances the versatility of AI technology and brings a new AI research and development paradigm. For the majority of developers, Based on the pre-trained large model, it is possible to develop a better AI model for the scene at a lower cost and with a low threshold." Wu Tian said.
The training and reasoning of Wenxin's large model rely on the support of the deep learning platform. At the same time, the large model, as an important member of the industrial-level model library in the Flying Paddle platform, has become an indispensable ability for the Flying Paddle platform to support AI innovation.
The challenges of large model training mainly come from "big", the large scale of model parameters, and the differences in the characteristics of different models and computing power platforms, which bring practical challenges to large model training. The distributed architecture of Flying Paddle takes these differences into consideration as a whole, uses an end-to-end adaptive distributed architecture, and automatically selects parallel strategies, automatically optimizes, and executes efficiently according to the characteristics of the model and computing power platform. Efficiency is also taken into account. Its innovation in parallel training strategy is to support adaptive parallel training for heterogeneous hardware, create a large model training solution that combines framework, computing power and algorithm, and achieve end-to-end extreme performance optimization.
Compared with training, large model inference faces greater challenges. Efficient reasoning of large models is the key to the realization of industrial applications of large models. At the deployment level of large models, Fei Pao has launched a full-process deployment plan for compression, reasoning, and service of large models to help large models land better.
It first makes the model lightweight through precision lossless model compression technology, and then fully mobilizes computing resources through adaptive distributed reasoning technology. Finally, through large-scale service deployment, the large model can be truly applied. The overall solution is universal and extensible, and can widely support different types of model structures to achieve high-speed reasoning. Currently, it has supported real-time online applications of large models such as natural language understanding, dialogue, and cross-modal generation.
These efforts are all to make the large model closer to the industry and land in the industry, not just laboratory technology.
Up to now, the Wenxin model has been used in industries such as industry, energy, education, finance, communications, and media, such as parts quality inspection in the industrial field, transmission line inspection in the energy field, inspiration for writing in the education industry, and financial industry. Contract information extraction, etc., really help enterprises reduce costs and increase efficiency and stimulate innovation. At the same time, the Wenxin model is also fully applied to Internet products such as intelligent search, information flow, and smart speakers to improve the efficiency and effect of users' acquisition of information, knowledge and services.
In general, Wu Tian gave three key paths to support the landing of Fei Pao Wenxin's large-scale model industry: build a large-scale model system that is more suitable for the needs of the scene, provide tools and methods to support the application landing in the whole process, and build an openness that stimulates innovation. ecology. Part of this ecological construction is the Wenxin·Yangu community, whose goal is to allow more people to have zero-distance access to AI large-scale model technology and stimulate innovation and creativity.
Comments